Event Recap
Data enrichment workers who clean, label, and moderate large sets of data are essential to machine learning (ML). Yet these workers often face poor working conditions and few labor protections, which not only impacts the wellbeing of data enrichment workers, but also affects the quality of the data AI technology is built on.
Partnership on AI (PAI) has launched a set of Data Enrichment Sourcing Guidelines for AI organizations to use to develop best practices with a positive impact on the lives of data enrichment workers, along with an accompanying case study, Resources for AI Practitioners, and blog post. We recently held a fascinating discussion around the importance of these resources, and explored putting these resources into practice by PAI Partner DeepMind.
This panel discussion moderated by Sonam Jindal, Program Lead for AI, Labor and the Economy unpacked why companies should be prioritizing responsible data enrichment practices, what this accomplishes, and what more we need to do, and through a real-world exploration of PAI Partner DeepMind’s process, challenges, and impact of putting the Guidelines into practice.
Speakers
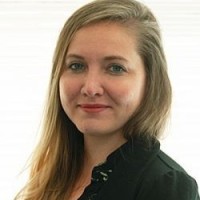
Jessica Custer
Associate Director, Equity, Inclusion, & Justice
BSR
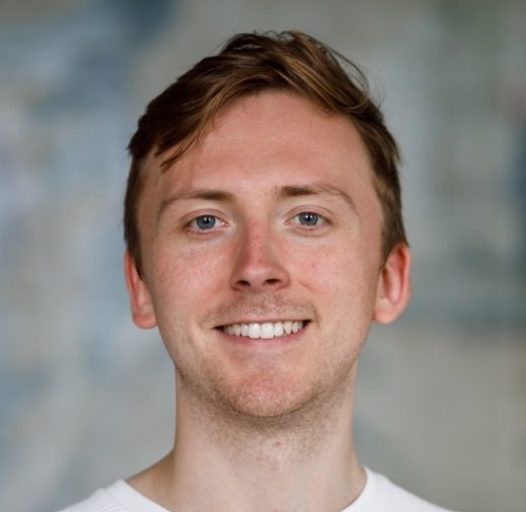
Will Hawkins
Operational Ethics and Safety Lead
DeepMind
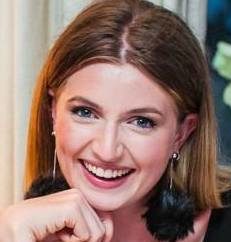
Antonia Paterson
Responsible Development and Innovation Manager
DeepMind
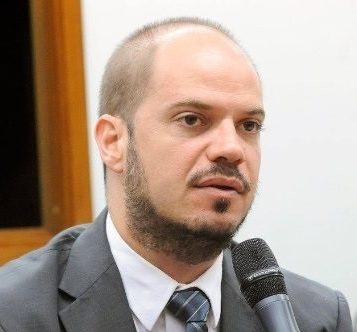
Jonas Valente
Cloudwork Postdoctoral Researcher Fairwork Foundation
Oxford Internet Institute
Moderator
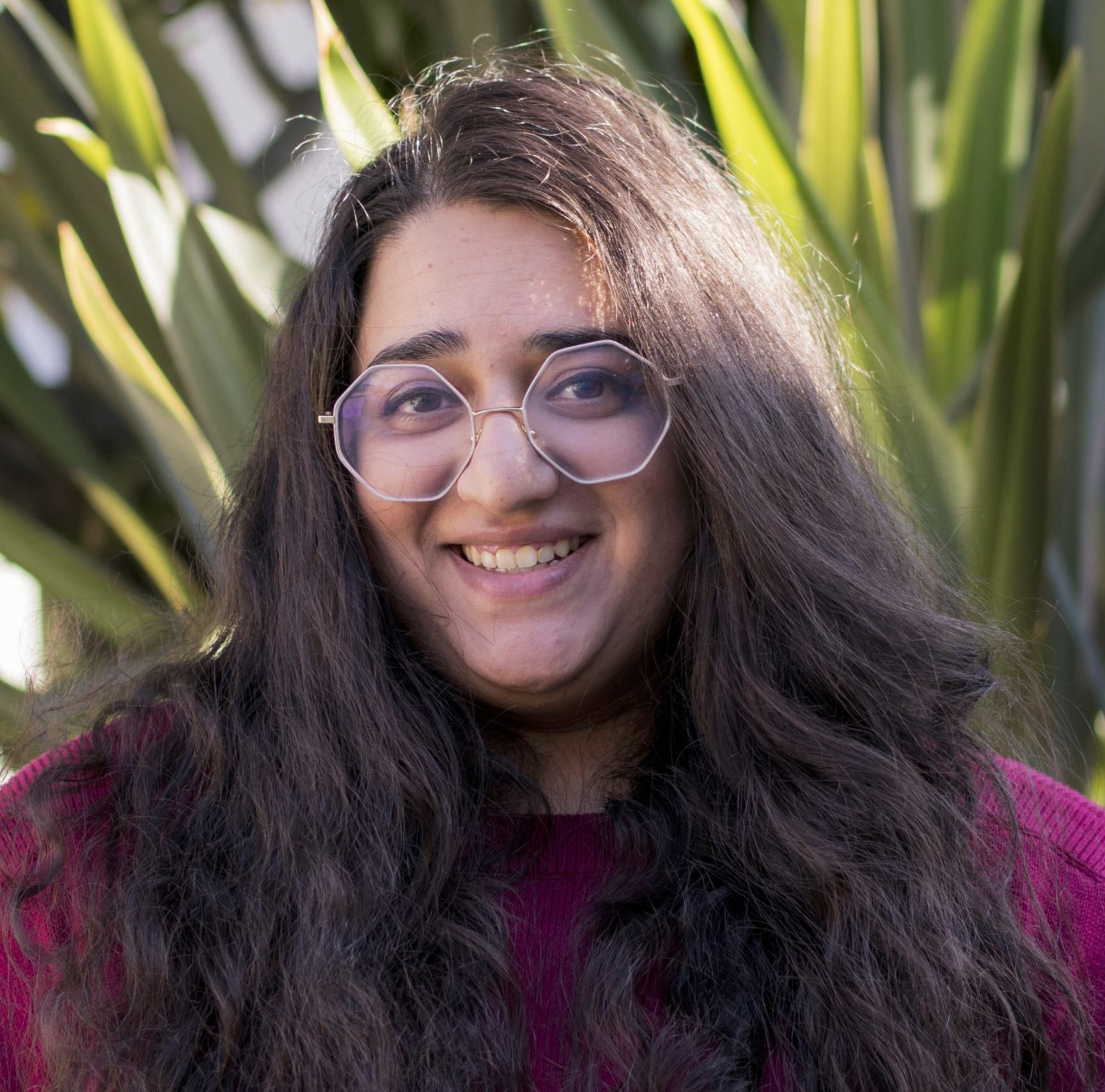
Sonam Jindal
Program Lead for AI, Labor and the Economy
Partnership on AI
Audience Questions
Question: What didn’t work about applying REC guidelines to use cases for data enrichment that seem to be working (for some aspects) for university researchers paying annotators working on datasets vis-a-vis a human subjects research framework?
Will: We spoke with various groups working with universities (contributing to this report from the Ada Lovelace institute which outlines some of the challenges). I also conducted some research which found that leading ML research involving data enrichment tasks via crowdsourcing was not engaging with IRB/REC processes (in industry or academia). The gap we found was that many researchers argue that data enrichment tasks (in research we see this in cases of labeling, evaluation, or production tasks) do not meet the definition provided by the US Common Rule, and therefore not in scope for IRB review (see recent discussion of this in Kaushik et al.). We also found that IRBs were not comfortable with reviewing data enrichment projects which involved employment contracts, because it impacted the ability for participants to engage in study without consequence (i.e. in contracted data enrichment projects, it is possible that a worker could lose their job for underperformance). These factors made us consider different approaches, and led us to the creation of the best practices and associated process (which works in parallel with our IRB process).
Question: As part of the ecosystem, what is the role of end users/consumers in the value chain?
Jessica: There is a history of end users / consumers playing an important role in raising awareness and expectations on issues they care about and ‘voting with their dollars’ for products and services that align with their values. And greater awareness and acknowledgement that human workers provide critical data enrichment services that power AI is a key part of catalyzing greater attention paid to the associated labor rights risks and concerns. So far, however, end consumers don’t seem to be a strong lever for awareness and change in this ecosystem since the B2B nature of data enrichment services make them further removed from an end consumer. That said, customers (e.g., the businesses that use AI powered products and services) alongside investors, governments, researchers, and other stakeholders (like workers themselves, and workers’ organizations) have clear and influential roles to play.
Question: To what extent has, or could, the AI industry learn from other sectors – for example agricultural and garment sectors – about the calculation of fair wages, working conditions and supplier monitoring?
Jessica: Definitely agree there is a lot of opportunity for the AI industry to learn from the journey that many other industries have taken with ‘responsible sourcing’ including to inform effective approaches and, importantly, to leapfrog missteps. Lessons on specific issues (like wages, precarity of contract work, etc.), processes for identifying and monitoring salient risks and partnering with suppliers to address them, and the opportunities for individual and collaborative action could be taken from e.g., the garment industry, electronics manufacturing, agriculture, business process outsourcing, etc., with acknowledgement of the key ecosystem differences (e.g., the digital and global nature of the workforce, task-based contract work, etc.).