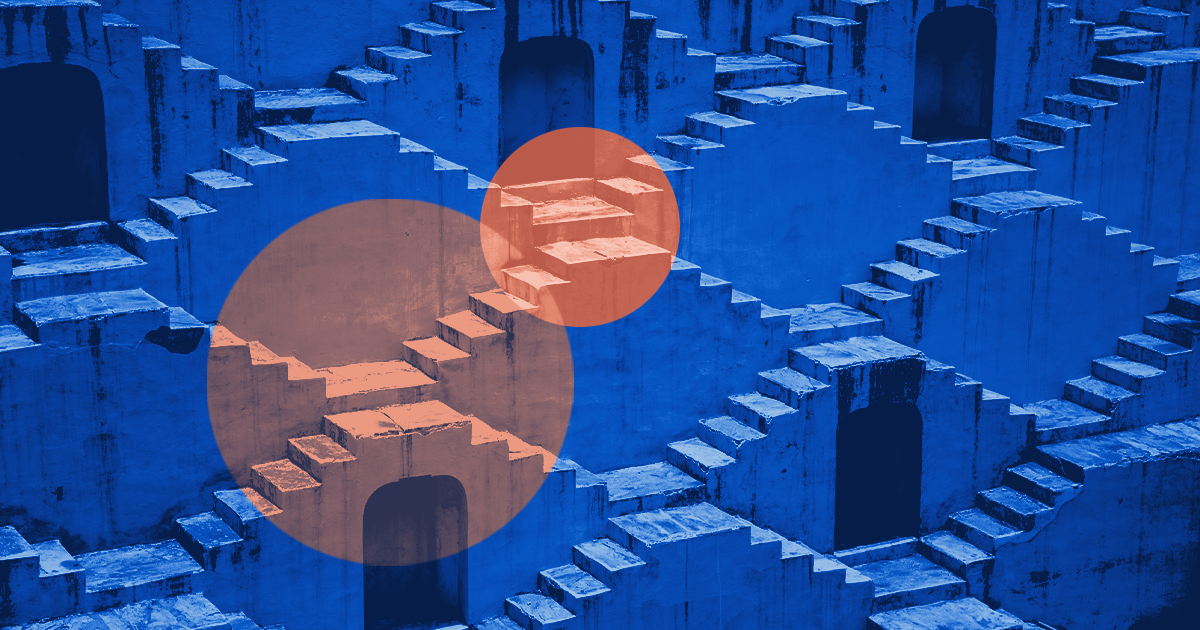
ABOUT ML Reference Document
Appendix C: Glossary
Appendix C: Glossary
API:
Application programming interface that defines the kinds of calls or requests that can be made, how to make them, the data formats that should be used, the conventions to follow, etc.
Autonomous vehicle:
A vehicle capable of sensing its environment and operating without human involvement.
Black box:
Any complex piece of equipment, typically a unit in an electronic system, with contents that are mysterious to the user.
Chatbot:
A computer program designed to simulate conversation with human users, especially over the internet.
Civil Society Organizations (CSOs):
Non-state, not-for-profit, voluntary entities formed by people in the social sphere that are separate from the State and the market. CSOs represent a wide range of interests and ties. They can include community-based organizations as well as non-governmental organizations (NGOs).
Disseminate:
To spread (something, especially information) widely.
Hyperparameter:
A parameter whose value is used to control the learning process.
Impacted non-users:
Persons or organizations impacted by a system even though they did not directly use it (i.e., a banking customer [nonuser] rejected for a home loan by a 3rd party algorithm [ML System] owned by some organization external to the bank and possibly implemented without the knowledge of the banking customer).
Industry norm:
An authoritative standard.
Intellectual property:
Any work or invention that is the result of creativity, such as a manuscript or a design, to which one has rights and for which one may apply for a patent, copyright, trademark, etc.
Iterative process:
A series of steps that are repeated, tweaking and improving the product with each repetition.
Model card:
A type of documentation artifact accompanying a given model that details the model itself, its intended uses, potential limitations, training parameters, datasets used, experimental information, and model evaluation results. (Definition from Hugging Face)
Multistakeholder process:
A process which aims to bring together the primary stakeholders such as businesses, civil society, governments, research institutions and non-government organizations to cooperate and participate in the dialogue, decision-making and implementation of solutions to common problems or goals.
NLP:
Natural Language Processing, or NLP for short, is broadly defined as the automatic manipulation of natural language, like speech and text, by software.
Objective and Key Result (OKR):
A collaborative goal-setting tool used by teams and individuals to set challenging, ambitious goals with measurable results. OKRs are how you track progress, create alignment, and encourage engagement around measurable goals
Reidentification risk:
The practice of matching anonymous data (also known as de-identified data) with publicly available information, or auxiliary data, in order to discover the individual to which the data belong to. This is a concern because companies with privacy policies, health care providers, and financial institutions may release the data they collect after the data has gone through the de-identification process.
Reproducibility:
The extent to which consistent results are obtained when an experiment is repeated.
Trade secrets:
Defined by the United States Patent and Trademark Office as information that has either actual or potential independent economic value by virtue of not being generally known, has value to others who cannot legitimately obtain the information, and is subject to reasonable efforts to maintain its secrecy.
ABOUT ML Reference Document
Section 0: How to Use this Document
Recommended Reading Plan
Quick Guides
How We Define
Contact for Support
Section 1: Project Overview
1.1 Statement of Importance for ABOUT ML Project
1.1.0 Importance of Transparency: Why a Company Motivated by the Bottom Line Should Adopt ABOUT ML Recommendations
1.1.1 About This Document and Version Numbering
1.1.2 ABOUT ML Goals and Plan
1.1.3 ABOUT ML Project Process and Timeline Overview
1.1.4 Who Is This Project For?
1.1.4.1 Audiences for the ABOUT ML Resources
1.1.4.2 Stakeholders That Should Be Consulted While Putting Together ABOUT ML Resources
1.1.4.3 Audiences for ABOUT ML Documentation Artifacts
1.1.4.4 Whose Voices Are Currently Reflected in ABOUT ML?
1.1.4.5 Origin Story
Section 2: Literature Review (Current Recommendations on Documentation for Transparency in the ML Lifecycle)
2.1 Demand for Transparency and AI Ethics in ML Systems
2.2 Documentation to Operationalize AI Ethics Goals
2.2.1 Documentation as a Process in the ML Lifecycle
2.2.2 Key Process Considerations for Documentation
2.3 Research Themes on Documentation for Transparency
2.3.1 System Design and Set Up
2.3.2 System Development
2.3.3 System Deployment
Section 3: Preliminary Synthesized Documentation Suggestions
3.4.1 Suggested Documentation Sections for Datasets
3.4.1.1 Data Specification
3.4.1.1.1 Motivation
3.4.1.2 Data Curation
3.4.1.2.1 Collection
3.4.1.2.2 Processing
3.4.1.2.3 Composition
3.4.1.2.4 Types and Sources of Judgement Calls
3.4.1.3 Data Integration
3.4.1.3.1 Use
3.4.1.3.2 Distribution
3.4.1.4 Maintenance
3.4.2 Suggested Documentation Sections for Models
3.4.2.1 Model Specifications
3.4.2.2 Model Training
3.4.2.3 Evaluation
3.4.2.4 Model Integration
3.4.2.5 Maintenance
Section 4: Current Challenges of Implementing Documentation
Section 5: Conclusions
Version 0
Version 1
Appendix A: Compiled List of Documentation Questions
Fact Sheets (Arnold et al. 2018)
Data Sheets (Gebru et al. 2018)
Model Cards (Mitchell et al. 2018)
A “Nutrition Label” for Privacy (Kelley et al. 2009)
The Dataset Nutrition Label: A Framework To Drive Higher Data Quality Standards (Holland et al. 2019)
Data Statements for Natural Language Processing: Toward Mitigating System Bias and Enabling Better Science (Bender and Friedman 2018)
Appendix B: Diverse Voices Process and Artifacts
Procurement Recruitment Email
Procurement Confirmation Email
Appendix C: Glossary
Sources Cited
- Holstein, K., Vaughan, J.W., Daumé, H., Dudík, M., u0026amp; Wallach, H.M. (2018). Improving Fairness in Machine Learning Systems: What Do Industry Practitioners Need? CHI.
- Young, M., Magassa, L. and Friedman, B. (2019) Toward inclusive tech policy design: a method for underrepresented voices to strengthen tech policy documents. Ethics and Information Technology 21(2), 89-103.
- World Wide Web Consortium Process Document (W3C) process outlined here: https://www.w3.org/2019/Process-20190301/
- Internet Engineering Task Force (IETF) process outlined here: https://www.ietf.org/standards/process/
- The Web Hypertext Application Technology Working Group (WHATWG) process outlined here: https://whatwg.org/faq#process
- Oever, N., Moriarty, K. The Tao of IETF: A novice's guide to the Internet Engineering Task Force. https://www.ietf.org/about/participate/tao/.
- Young, M., Magassa, L. and Friedman, B. (2019) Toward inclusive tech policy design: a method for underrepresented voices to strengthen tech policy documents. Ethics and Information Technology 21(2), 89-103.
- Friedman, B, Kahn, Peter H., and Borning, A., (2008) Value sensitive design and information systems. In Kenneth Einar Himma and Herman T. Tavani (Eds.) The Handbook of Information and Computer Ethics., (pp. 70-100) John Wiley u0026amp; Sons, Inc. http://jgustilo.pbworks.com/f/the-handbook-of-information-and-computer-ethics.pdf#page=104; Davis, J., and P. Nathan, L. (2015). Value sensitive design: applications, adaptations, and critiques. Handbook of Ethics, Values, and Technological Design: Sources, Theory, Values and Application Domains. (pp. 11-40) DOI: 10.1007/978-94-007-6970-0_3. https://www.researchgate.net/publication/283744306_Value_Sensitive_Design_Applications_Adaptations_and_Critiques; Borning, A. and Muller, M. (2012). Next steps for value sensitive design. In Proceedings of the SIGCHI Conference on Human Factors in Computing Systems (CHI '12). (pp 1125-1134) DOI: https://doi.org/10.1145/2207676.2208560 https://dl.acm.org/citation.cfm?id=2208560
- Pichai, S., (2018). AI at Google: our principles. The Keyword. https://www.blog.google/technology/ai/ai-principles/; IBM’s Principles for Trust and Transparency. IBM Policy. https://www.ibm.com/blogs/policy/trust-principles/; Microsoft AI principles. Microsoft. https://www.microsoft.com/en-us/ai/our-approach-to-ai; Ethically Aligned Design – Version II. IEEE. https://standards.ieee.org/content/dam/ieee-standards/standards/web/documents/other/ead_v2.pdf
- Zeng, Y., Lu, E., and Huangfu, C. (2018) Linking artificial intelligence principles. CoRR https://arxiv.org/abs/1812.04814.
- essica Fjeld, Hannah Hilligoss, Nele Achten, Maia Levy Daniel, Sally Kagay, and Joshua Feldman, (2018). Principled artificial intelligence - a map of ethical and rights based approaches, Berkman Center for Internet and Society, https://ai-hr.cyber.harvard.edu/primp-viz.html
- Jobin, A., Ienca, M., u0026amp; Vayena, E. (2019). Artificial Intelligence: the global landscape of ethics guidelines. arXiv preprint arXiv:1906.11668. https://arxiv.org/pdf/1906.11668.pdf
- Jobin, A., Ienca, M., u0026amp; Vayena, E. (2019). Artificial Intelligence: the global landscape of ethics guidelines. arXiv preprint arXiv:1906.11668. https://arxiv.org/pdf/1906.11668.pdf
- Ananny, M., and Kate Crawford (2018). Seeing without knowing: Limitations of the transparency ideal and its application to algorithmic accountability. New Media and Society 20 (3): 973-989.
- Whittlestone, J., Nyrup, R., Alexandrova, A., u0026amp; Cave, S. (2019, January). The Role and Limits of Principles in AI Ethics: Towards a Focus on Tensions. In Proceedings of the AAAI/ACM Conference on AI Ethics and Society, Honolulu, HI, USA (pp. 27-28). http://www.aies-conference.com/wp-content/papers/main/AIES-19_paper_188.pdf; Mittelstadt, B. (2019). AI Ethics–Too Principled to Fail? https://papers.ssrn.com/sol3/papers.cfm?abstract_id=3391293
- Greene, D., Hoffmann, A. L., u0026amp; Stark, L. (2019, January). Better, nicer, clearer, fairer: A critical assessment of the movement for ethical artificial intelligence and machine learning. In Proceedings of the 52nd Hawaii International Conference on System Sciences. https://scholarspace.manoa.hawaii.edu/handle/10125/59651
- Raji, I. D., u0026amp; Buolamwini, J. (2019). Actionable auditing: Investigating the impact of publicly naming biased performance results of commercial ai products. In AAAI/ACM Conf. on AI Ethics and Society (Vol. 1). https://www.media.mit.edu/publications/actionable-auditing-investigating-the-impact-of-publicly-naming-biased-performance-results-of-commercial-ai-products/
- Algorithmic Impact Assessment (2019) Government of Canada https://www.canada.ca/en/government/system/digital-government/modern-emerging-technologies/responsible-use-ai/algorithmic-impact-assessment.html
- Benjamin, M., Gagnon, P., Rostamzadeh, N., Pal, C., Bengio, Y., u0026amp; Shee, A. (2019). Towards Standardization of Data Licenses: The Montreal Data License. arXiv preprint arXiv:1903.12262. https://arxiv.org/abs/1903.12262; Responsible AI Licenses v0.1. RAIL: Responsible AI Licenses. https://www.licenses.ai/ai-licenses
- See Citation 5
- Safe Face Pledge. https://www.safefacepledge.org/; Montreal Declaration on Responsible AI. Universite de Montreal. https://www.montrealdeclaration-responsibleai.com/; The Toronto Declaration: Protecting the right to equality and non-discrimination in machine learning systems. (2018). Amnesty International and Access Now. https://www.accessnow.org/cms/assets/uploads/2018/08/The-Toronto-Declaration_ENG_08-2018.pdf ; Dagsthul Declaration on the application of machine learning and artificial intelligence for social good. https://www.dagstuhl.de/fileadmin/redaktion/Programm/Seminar/19082/Declaration/Declaration.pdf
- Dobbe, R., Dean, S., Gilbert, T., u0026amp; Kohli, N. (2018). A Broader View on Bias in Automated Decision-Making: Reflecting on Epistemology and Dynamics. https://arxiv.org/pdf/1807.00553.pdf
- Wagstaff, K. (2012). Machine learning that matters. https://arxiv.org/pdf/1206.4656.pdf ; Friedman, B., Kahn, P. H., Borning, A., u0026amp; Huldtgren, A. (2013). Value sensitive design and information systems. In Early engagement and new technologies: Opening up the laboratory (pp. 55-95). Springer, Dordrecht. https://vsdesign.org/publications/pdf/non-scan-vsd-and-information-systems.pdf
- Dobbe, R., Dean, S., Gilbert, T., u0026amp; Kohli, N. (2018). A Broader View on Bias in Automated Decision-Making: Reflecting on Epistemology and Dynamics. https://arxiv.org/pdf/1807.00553.pdf
- Safe Face Pledge. https://www.safefacepledge.org/
- Montreal Declaration on Responsible AI. Universite de Montreal. https://www.montrealdeclaration-responsibleai.com/
- Diverse Voices How To Guide. Tech Policy Lab, University of Washington. https://techpolicylab.uw.edu/project/diverse-voices/
- Bender, E. M., u0026amp; Friedman, B. (2018). Data statements for natural language processing: Toward mitigating system bias and enabling better science. Transactions of the Association for Computational Linguistics, 6, 587-604.
- Ethically Aligned Design – Version II. IEEE. https://standards.ieee.org/content/dam/ieee-standards/standards/web/documents/other/ead_v2.pdf
- Gebru, T., Morgenstern, J., Vecchione, B., Vaughan, J. W., Wallach, H., Daumeé III, H., u0026amp; Crawford, K. (2018). Datasheets for datasets. https://arxiv.org/abs/1803.09010 https://arxiv.org/abs/1803.09010; Hazard Communication Standard: Safety Data Sheets. Occupational Safety and Health Administration, US Department of Labor. https://www.osha.gov/Publications/OSHA3514.html
- Holland, S., Hosny, A., Newman, S., Joseph, J., u0026amp; Chmielinski, K. (2018). The dataset nutrition label: A framework to drive higher data quality standards. https://arxiv.org/abs/1805.03677; Kelley, P. G., Bresee, J., Cranor, L. F., u0026amp; Reeder, R. W. (2009). A nutrition label for privacy. In Proceedings of the 5th Symposium on Usable Privacy and Security (p. 4). ACM. http://cups.cs.cmu.edu/soups/2009/proceedings/a4-kelley.pdf
- Mitchell, M., Wu, S., Zaldivar, A., Barnes, P., Vasserman, L., Hutchinson, B., ... u0026amp; Gebru, T. (2019, January). Model cards for model reporting. In Proceedings of the Conference on Fairness, Accountability, and Transparency (pp. 220-229). ACM. https://arxiv.org/abs/1810.03993
- Hind, M., Mehta, S., Mojsilovic, A., Nair, R., Ramamurthy, K. N., Olteanu, A., u0026amp; Varshney, K. R. (2018). Increasing Trust in AI Services through Supplier's Declarations of Conformity. https://arxiv.org/abs/1808.07261
- Veale M., Van Kleek M., u0026amp; Binns R. (2018) ‘Fairness and Accountability Design Needs for Algorithmic Support in High-Stakes Public Sector Decision-Making’ in Proceedings of the ACM Conference on Human Factors in Computing Systems, CHI 2018. https://arxiv.org/abs/1802.01029.
- Benjamin, M., Gagnon, P., Rostamzadeh, N., Pal, C., Bengio, Y., u0026amp; Shee, A. (2019). Towards Standardization of Data Licenses: The Montreal Data License. https://arxiv.org/abs/1903.12262
- Cooper, D. M. (2013, April). A Licensing Approach to Regulation of Open Robotics. In Paper for presentation for We Robot: Getting down to business conference, Stanford Law School.
- Responsible AI Practices. Google AI. https://ai.google/education/responsible-ai-practices
- Everyday Ethics for Artificial Intelligence. (2019). IBM. https://www.ibm.com/watson/assets/duo/pdf/everydayethics.pdf
- Federal Trade Commission. (2012). Best Practices for Common Uses of Facial Recognition Technologies (Staff Report). Federal Trade Commission, 30. https://www.ftc.gov/sites/default/files/documents/reports/facing-facts-best-practices-common-uses-facial-recognition-technologies/121022facialtechrpt.pdf
- Microsoft (2018). Responsible bots: 10 guidelines for developers of conversational AI. https://www.microsoft.com/en-us/research/uploads/prod/2018/11/Bot_Guidelines_Nov_2018.pdf
- Tramer, F., Atlidakis, V., Geambasu, R., Hsu, D., Hubaux, J. P., Humbert, M., ... u0026amp; Lin, H. (2017, April). FairTest: Discovering unwarranted associations in data-driven applications. In 2017 IEEE European Symposium on Security and Privacy (EuroSu0026amp;P) (pp. 401-416). IEEE. https://github.com/columbia/fairtest, https://www.mhumbert.com/publications/eurosp17.pdf
- Kishore Durg (2018). Testing AI: Teach and Test to raise responsible AI. Accenture Technology Blog. https://www.accenture.com/us-en/insights/technology/testing-AI
- Kush R. Varshney (2018). Introducing AI Fairness 360. IBM Research Blog. https://www.ibm.com/blogs/research/2018/09/ai-fairness-360/
- Dave Gershgorn (2018). Facebook says it has a tool to detect bias in its artificial intelligence. Quartz. https://qz.com/1268520/facebook-says-it-has-a-tool-to-detect-bias-in-its-artificial-intelligence/
- James Wexler. (2018) The What-If Tool: Code-Free Probing of Machine Learning Models. Google AI Blog. https://ai.googleblog.com/2018/09/the-what-if-tool-code-free-probing-of.html
- Miro Dudík, John Langford, Hanna Wallach, and Alekh Agarwal (2018). Machine Learning for fair decisions. Microsoft Research Blog. https://www.microsoft.com/en-us/research/blog/machine-learning-for-fair-decisions/
- Veale, M., Binns, R., u0026amp; Edwards, L. (2018). Algorithms that Remember: Model Inversion Attacks and Data Protection Law. Phil. Trans. R. Soc. A, 376, 20180083. https://doi.org/10/gfc63m
- Floridi, L. (2010, February). Information: A Very Short Introduction.
- Data Information Specialists Committee UK, 2007. http://www.disc-uk.org/qanda.html.
- Harwell, Drew. “Federal Study Confirms Racial Bias of Many Facial-Recognition Systems, Casts Doubt on Their Expanding Use.” The Washington Post, WP Company, 21 Dec. 2019, www.washingtonpost.com/technology/2019/12/19/federal-study-confirms-racial-bias-many-facial-recognition-systems-casts-doubt-their-expanding-use/
- Hildebrandt, M. (2019) ‘Privacy as Protection of the Incomputable Self: From Agnostic to Agonistic Machine Learning’, Theoretical Inquiries in Law, 20(1) 83–121.
- D'Amour, A., Heller, K., Moldovan, D., Adlam, B., Alipanahi, B., Beutel, A., ... u0026amp; Sculley, D. (2020). Underspecification presents challenges for credibility in modern machine learning. arXiv preprint arXiv:2011.03395.
- Selinger, E. (2019). ‘Why You Can’t Really Consent to Facebook’s Facial Recognition’, One Zero. https://onezero.medium.com/why-you-cant-really-consent-to-facebook-s-facial-recognition-6bb94ea1dc8f
- Lum, K., u0026amp; Isaac, W. (2016). To predict and serve?. Significance, 13(5), 14-19. https://rss.onlinelibrary.wiley.com/doi/full/10.1111/j.1740-9713.2016.00960.x
- LabelInsight (2016). “Drive Long-Term Trust u0026amp; Loyalty Through Transparency”. https://www.labelinsight.com/Transparency-ROI-Study
- Crawford and Paglen, https://www.excavating.ai/
- Geva, Mor u0026amp; Goldberg, Yoav u0026amp; Berant, Jonathan. (2019). Are We Modeling the Task or the Annotator? An Investigation of Annotator Bias in Natural Language Understanding Datasets. https://arxiv.org/pdf/1908.07898.pdf
- Bender, E. M., u0026amp; Friedman, B. (2018). Data statements for natural language processing: Toward mitigating system bias and enabling better science. Transactions of the Association for Computational Linguistics, 6, 587-604.
- Desmond U. Patton et al (2017).
- See Cynthia Dwork et al.,
- Katta Spiel, Oliver L. Haimson, and Danielle Lottridge. (2019). How to do better with gender on surveys: a guide for HCI researchers. Interactions. 26, 4 (June 2019), 62-65. DOI: https://doi.org/10.1145/3338283
- A. Doan, A. Y. Halevy, and Z. G. Ives. Principles of Data Integration. Morgan Kaufmann, 2012
- Momin M. Malik. (2019). Can algorithms themselves be biased? Medium. https://medium.com/berkman-klein-center/can-algorithms-themselves-be-biased-cffecbf2302c
- Fire, Michael, and Carlos Guestrin (2019). “Over-Optimization of Academic Publishing Metrics: Observing Goodhart’s Law in Action.” GigaScience 8 (giz053). https://doi.org/10.1093/gigascience/giz053.
- Vogelsang, A., u0026amp; Borg, M. (2019, September). Requirements engineering for machine learning: Perspectives from data scientists. In 2019 IEEE 27th International Requirements Engineering Conference Workshops (REW) (pp. 245-251). IEEE
- Eckersley, P. (2018). Impossibility and Uncertainty Theorems in AI Value Alignment (or why your AGI should not have a utility function). arXiv preprint arXiv:1901.00064.
- Partnership on AI. Report on Algorithmic Risk Assessment Tools in the U.S. Criminal Justice System, Requirement 5.
- Eckersley, P. (2018). Impossibility and Uncertainty Theorems in AI Value Alignment (or why your AGI should not have a utility function). arXiv preprint arXiv:1901.00064.https://arxiv.org/abs/1901.00064
- If it is not, there is likely a bug in the code. Checking a predictive model's performance on the training set cannot distinguish irreducible error (which comes from intrinsic variance of the system) from error introduced by bias and variance in the estimator; this is universal, and has nothing to do with different settings or
- Selbst, Andrew D. and Boyd, Danah and Friedler, Sorelle and Venkatasubramanian, Suresh and Vertesi, Janet (2018). “Fairness and Abstraction in Sociotechnical Systems”, ACM Conference on Fairness, Accountability, and Transparency (FAT*). https://ssrn.com/abstract=3265913
- Tools that can be used to explore and audit the predictive model fairness include FairML, Lime, IBM AI Fairness 360, SHAP, Google What-If Tool, and many others
- Wagstaff, K. (2012). Machine learning that matters. arXiv preprint arXiv:1206.4656. https://arxiv.org/abs/1206.4656