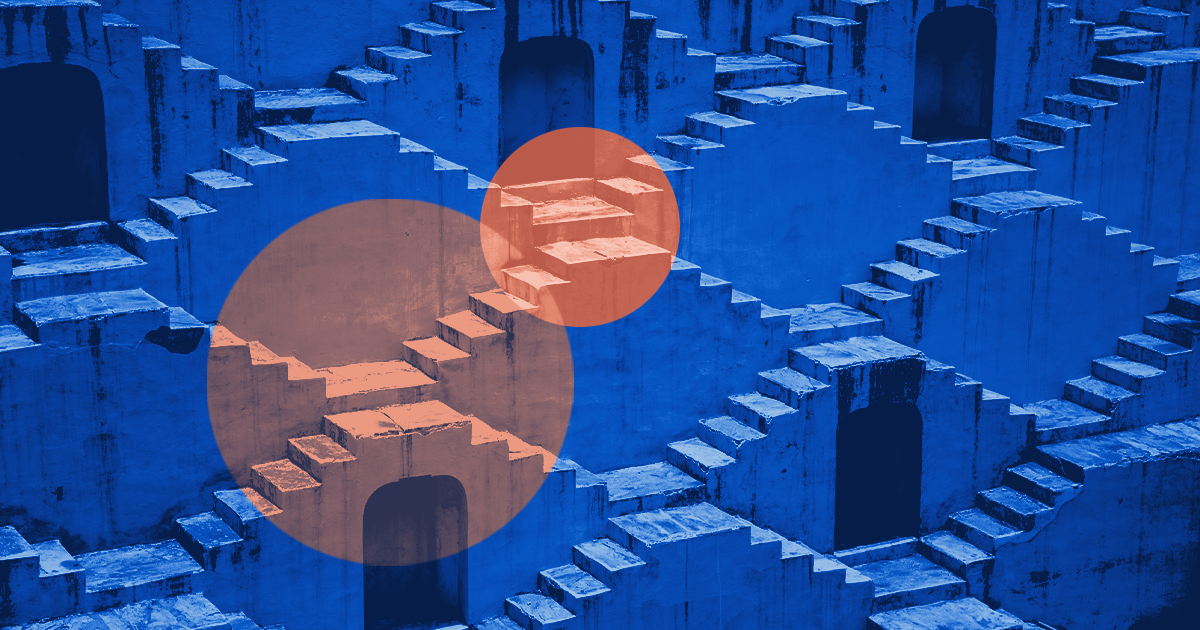
ABOUT ML Reference Document
3.4.1.3 Data Integration
3.4.1.3 Data Integration
Data Integration
We use the tenets of the Doan et. al (2012) definition which infers that proper data integration offers “uniform access to a set of autonomous and heterogeneous data sources.”
The task is often challenging for system logical, social, and administrative reasons.
Data integration might mean/include a few things: connecting data sources, data distribution to users, inclusion of data pipeline and data management, maintenance of data, audit of data usage and issues. It might be useful to separate the technical and data science aspect from the safety and continuous use of data.
3.4.1.3.1 Use
3.4.1.3.1 Use
Stating the intended and permitted uses of a dataset can be helpful for users to understand whether the dataset is appropriate for their projects. In particular, such disclosures should include information on how the composition of the dataset or way in which it was collected and cleaned might affect future uses, including what the data can and cannot represent, what applications and conclusions are not appropriate to draw, and what types of consent was obtained from data subjects. This is also the section to discuss guardrails on data use to avoid re-identification through certain kinds of data joins. Links to existing literature that uses the dataset can be helpful for illustrative purposes. This section would also include information on uses to be avoided and explanations of possible adverse consequences that might result from inappropriate uses. For data sets with high potential for misuse, this section may instead list the acceptable uses for the dataset and forbid all others. In the future, we may explore how documentation recommendations fit in with ongoing data licensing projects.A. Doan, A. Y. Halevy, and Z. G. Ives. Principles of Data Integration. Morgan Kaufmann, 2012
Pros/Cons
Some advantages to these disclosures are that information about the intent of the dataset helps give potential users greater context and minimizes potential misuse. It is important to clarify common misconceptions. For example, data collected to classify people’s facial expressions (smiling, frowning, etc.) might not be appropriate to use to classify people’s underlying moods (happiness, sadness, etc.). This information also would help hold users of the dataset accountable.
Some challenges to making these disclosures include that it is difficult to identify all potential malicious uses of a dataset. In addition, malicious actors might purposefully use a dataset in improper ways. Cautious legal departments may also be concerned about the possibility for liability with disclosing appropriate and inappropriate uses of the data. This can be mitigated through consultation with legal counsel, although that may be impractical where data sources include the public and are used at a high frequency (e.g., capturing visual data in airports). Explorations related to the following research questions could uncover insights into barriers to implementation along with mitigation strategies to overcome those barriers.
Sample Documentation Questions
- Is there a repository that links to any or all papers or systems that use the dataset? (Gebru et al. 2018)
- Are there tasks for which the dataset should not be used? (Gebru et al. 2018)
- Was there consent obtained by data subjects, and does that consent place limits on the use of the data?
3.4.1.3.2 Distribution
3.4.1.3.2 Distribution
Distribution disclosures should relay how the dataset’s creators will distribute the data for use and update the data, either internally between segments of their company or publically. This makes it easier for people to find and use the data and clarifies the intended audience. Such disclosures should include information about the accessibility of the dataset and the intended audience. Licensing and the timing of licenses and consent are important considerations in this disclosure process. Depending on how broadly the dataset is distributed and the original consent given, additional consent might need to be obtained from the subjects of the data. Specific information on recipients may also need to be provided as a matter of local law. The distribution process should involve appropriate steps to preserve the privacy of data subjects. For example, there might be a log-in needed to access the dataset and dataset users might need to sign a contract stipulating the conditions of use. This would have the added benefit of ensuring that if someone withdrew their consent to have their data included in the dataset, their data could be deleted in one place. However, if the data is downloaded or stored by another user, consent revocation becomes more difficult to manage, and future tools need to be developed to manage this process. In addition, the dataset should be transformed such that the reidentification risk is permissible in line with relevant legal regimes prior to distribution and safeguards should be put in place to prevent de-anonymization.
Sample Documentation Questions
- How will the dataset will be distributed (e.g., tarball on website, API, GitHub)? Does the dataset have a digital object identifier (DOI)? (Gebru et al. 2018)
- Will the dataset be distributed under a copyright or other intellectual property (IP) license and/or under applicable terms of use (ToU)? (Gebru et al. 2018)
- Do any export controls or other regulatory restrictions apply to the dataset or to individual instances? (Gebru et al. 2018)
- What documentation and metadata will you be releasing with the model? (Gebru et al. 2018)
3.4.1.4 Maintenance
3.4.1.4 Maintenance
Providing information about the maintenance of datasets is important for helping users know whether they are using the latest dataset and whether the dataset will be kept up to date. The default assumption is generally that datasets are not maintained. If the dataset is not maintained, however, there can be concerns about the interpretability and applicability of the dataset for new projects. Developers who are interested in using the data should be informed about these potential issues so that they can draw appropriate inferences.
GDPR
Moreover, if the dataset is not maintained, it can be difficult for individuals to remove their data. This is especially an issue with criminal records, which may need to be expunged periodically depending on local law. For developers for the EU, this can also create complications with GDPR, the overarching principle of accuracy, and the rights to rectification and to be forgotten (“right to erasure”). To ensure that users are using the latest version of the dataset, measures can be taken to ensure that users cannot download past versions of a dataset or that the dataset has an expiration date after which it is unusable without being updated. However, such measures could prevent comparisons of how different machine learning systems work on old versus new datasets.
Pros/Cons
Some benefits are that users would be able to better understand why and how the dataset changed. Proper maintenance techniques also make it possible for individuals to remove their content if they want to.
Some disadvantages are that maintaining datasets can be time- and resource-intensive and being explicit about plans for maintenance does, to some extent, require the dataset developer to follow through. In addition, doing maintenance well can be difficult, as there are potential issues with versioning and shifts in technology. Further, completely eliminating older datasets (as opposed to simply marking them as obsolete) can prevent historical analysis of how datasets have changed over time. Explorations related to the following research questions could uncover insights into barriers to implementation as well as meaningful concrete examples of GDPR relevance to ML documentation.
Sample Documentation Questions
- Is there an erratum (list of mistakes)? (Gebru et al. 2018)
- Will the dataset be updated? If so, how often, by whom, and how will updates be communicated to users? (Gebru et al. 2018)
- When will the dataset expire? Is there a set time limit after which the data should be considered obsolete?
Those attempting documentation practices within any phase of the machine learning lifecycle can consider how ethics approval might be customizable for different disciplines or change over time by paying particular attention to:
- Establishing ethics scores and approvals
- Developing clear objectives during data collection with benchmarks and constraints for review (i.e. in 5, 7, or 10 years)
- Ensuring ease of contact for participants to whom the data belongs, asking questions such as “If you are doing longitudinal processes with a fairly transient population, how do you ensure you can find that person later to re-establish consent? “ and “Is that information still relevant for our use?”
- Committing to, after finding issues with a dataset, discontinuing use and/or putting mitigation practices in place
- Considering your team’s ability to fix, update, or remove any model or data released from distribution
- Replacing problematic benchmarks and encouraging use of better alternatives