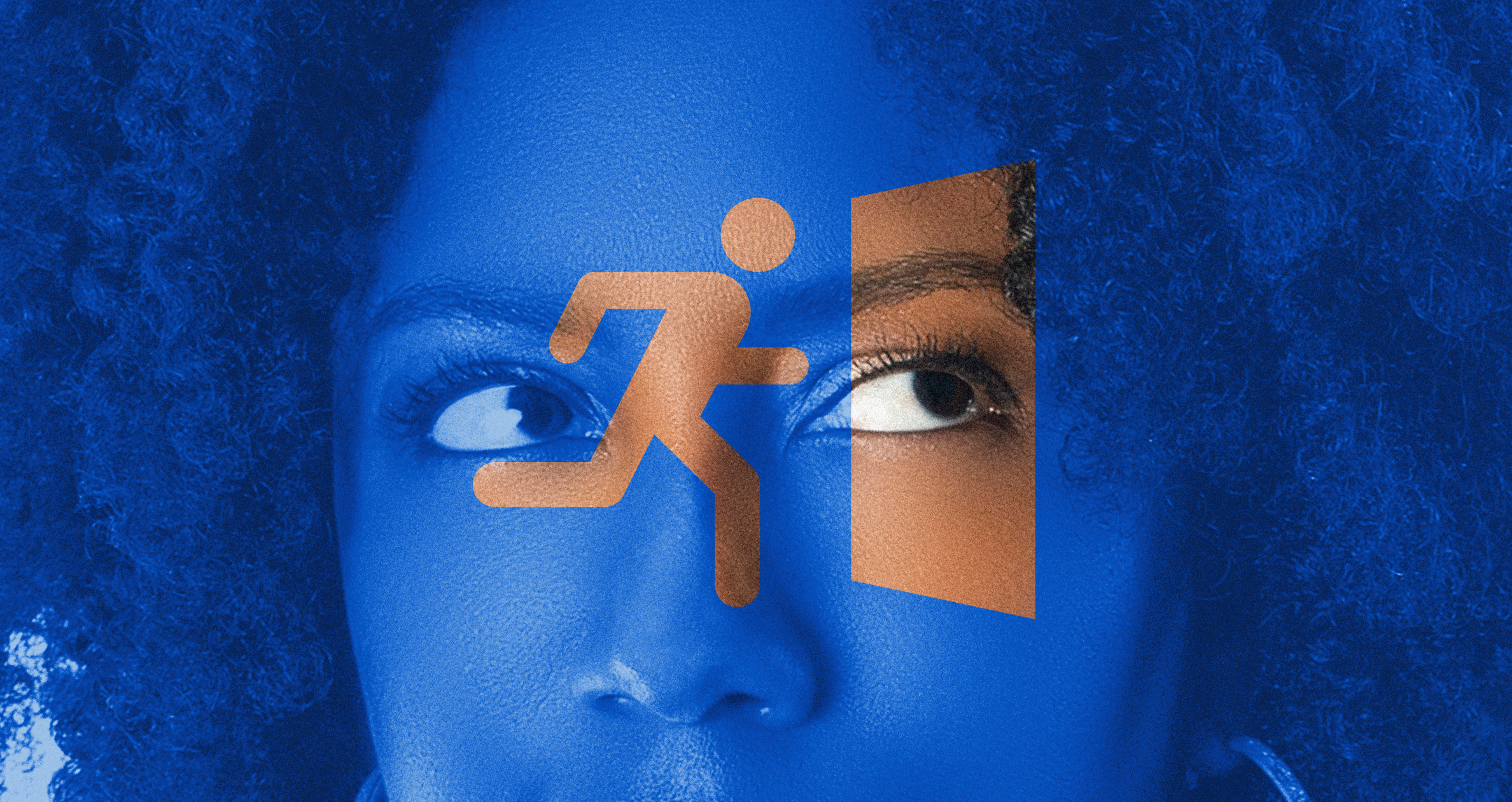
After the Offer: The Role of Attrition in AI’s ‘Diversity Problem’
Executive Summary
As a field, AI struggles to retain team members from diverse backgrounds. Given the far-reaching effects of algorithmic systems and the documented harms to marginalized communities, the fact that these communities are not represented on AI teams is particularly troubling. Why is this such a widespread phenomenon and what can be done to close the gap? This research paper, “After the Offer: The Role of Attrition in AI’s ‘Diversity Problem’” seeks to answer these questions, providing four recommendations for how organizations can make the AI field more inclusive. Click the button below to download a summary of these recommendations or continue on to read the paper in full.
Amid heightened attention to society-wide racial and social injustice, organizations in the AI space have been urged to investigate the harmful effects that AI has had on marginalized populations. It’s an issue that engineers, researchers, project managers, and various leaders in both tech companies and civil society organizations have devoted significant time and resources to in recent years. In examining the effects of AI, organizations must consider who exactly has been designing these technologies.
Diversity reports have revealed that the people working at the organizations that develop and deploy AI lack diversity across several dimensions. While organizations have blamed pipeline problems in the past, research has increasingly shown that once workers belonging to minoritized identities get hired in these spaces, systemic difficulties affect their experiences in ways that their peers from dominant groups do not have to worry about.
Attrition in the tech industry is a problem that disproportionately affects minoritized workers. In AI, where technologies already have a disproportionately negative impact on these communities, this is especially troublesome.
Minoritized Workers
We are left wondering: What leads to these folks leaving their teams, organizations, or even the AI field more broadly? What about the AI field in particular influences these people to stay or leave? And what can organizations do to stem this attrition to make their environments more inclusive?
The current study uses interviews with folks belonging to minoritized identities across the AI field, managers, and DEI (diversity, equity, and inclusion)- leaders in tech to get rich information about what aspects of cultures within an organization promote inclusion or contribute to attrition. Themes that emerged during these interviews formed 3 key takeaways:
- Diversity makes for better team climates
- Systemic supports are difficult but necessary to undo the current harms to minoritized workers
- Individual efforts to change organizational culture fall disproportionately on minoritized folks who are usually not professionally rewarded for their efforts
In line with these takeaways, the study makes 4 recommendations about what can be done to make the AI field more inclusive for workers:
- Organizations must systemically support ERGs
- Organizations must intentionally diversify leadership and managers
- DEI trainings must be specific in order to be effective and be more connected to the content of AI work
- Organizations must interrogate their values as practiced and fundamentally alter them to include the perspectives of people who are not White, cis, or male
These takeaways and recommendations are explored in more depth below.
Key Takeaways
Key Takeaways
1. Diversity makes for better team climates
Across interviews, participants consistently expressed that managers who belonged to minoritized identities or who took the time to learn about working with diverse identities were more supportive of their needs and career goals. Such efforts reportedly resulted in teams that were also more diverse, inclusive, interdisciplinary, and engendering of a positive team culture/climate. In these environments, workers belonging to minoritized identities thrived. A diversity in backgrounds and perspectives was particularly important for AI teams that needed to solve interdisciplinary problems.
Conversely, the negative impact of work environments that were sexist or where participants experienced acts of prejudice such as microaggressions was also a recurring theme.
While collaborative or positive work environments were also a common theme, such environments did not in themselves negate predominant cultures which deprioritized “DEI-focused” work, work that was highly interdisciplinary, or work that did not serve the dominant group. Negative organizational cultures seemed to exacerbate experiences of prejudice or discrimination on AI teams.
2. Systemic supports are difficult but necessary to undo the current harms to minoritized workers
Participants belonging to minoritized identities said that they either left or intended to leave organizations that did not support their continued career growth or possessed values that did not align with their own. Consistent with this, participants described examples of their organizations not valuing the content of their work.
Participants also tied their desires to leave with instances of prejudice or discrimination, which may also be related to “toxic” work environments. Some participants reported instances of being tokenized or being subject to negative stereotypes about their identity groups, somewhat reflective of wider contexts in tech beyond AI.
Systemic supports include incentive structures that allow minoritized workers to succeed at every level, from the teams that they work with actively validating their experiences to their managers finding the best ways for them to deliver work products in accordance with both individual and institutional needs. Guidelines for promotion that recognize the barriers these workers face in environments mostly occupied by dominant group norms are another important support.
3. Individual efforts to change organizational culture fall disproportionately on minoritized folks who are usually not professionally rewarded for their efforts
Individuals discussed ways in which they tried to make their workplaces or teams more inclusive or otherwise sought to incorporate diverse perspectives into their work around AI. Participants sometimes had to contend with bias against DEI efforts, reporting that other workers in their organizations would dismiss their efforts as lacking rigor or focus on the product.
There were some institutional efforts to foster a more inclusive culture, most commonly DEI trainings. DEI trainings that were very specific to some groups (e.g., gender diverse folks, Black people) were reported as being the most effective. However, even when they were specific, DEI trainings seemed to be disconnected from some aspects of the workplace climate or the content of what teams were working on.
Participants who mentioned Employee Resource Groups (ERGs) uniformly praised them, discussing the huge positive impact they had on a personal level, forming the bases of their social support networks in their organizations and having a strong impact on their ability to integrate aspects of their identities or other “DEI topics” they were passionate about into their work.
Recommendations
Recommendations
1. Organizations must systemically support ERGs
Employees specifically named ERGs as one of their main sources of support even in work environments that were otherwise toxic.. Additionally, ERGs provided built-in mentorship for those who did not have ready access to mentors or whose supervisors had not done the work to understand the kinds of support needed for those of minoritized identities to thrive in predominantly White and male environments.
Within these ERGs, there existed other grass-roots initiatives that supported workers, such as informal talking circles and networks of employees that essentially provided peer mentoring that participants found crucial to navigating White- and male-dominated spaces. The mentorship provided by ERGs was also essential when HR failed to provide systemic support for staff and instead prioritized protecting the organization.
While participants uniformly praised ERGs, they required large amounts of time from staff members that detracted from their work. Such groups also ran the risk of getting taken over by leadership and having their original mission derailed. Institutions should seek a balance between supporting these groups and giving them the freedom to organize in pursuit of their own best interests.
ERGs will not necessarily make an organization’s AI or tech more inclusive. Rather, systematically supporting ERGs will provide more support and community for minoritized workers, which is meant to promote a more inclusive workplace in general.
2. Organizations must intentionally diversify leadership and managers
Participants repeatedly pointed to managers and upper-level leaders who belonged to minoritized identities (especially racial ones) as important influences, changing policy that permeated through various levels of their organizations. A diverse workforce may also bring with it multiple perspectives, including those belonging to people from different disciplines who may be interested in working in the AI field due to the opportunity for interdisciplinary collaboration, research, and product development. Bringing in folks from various academic, professional, and technical backgrounds to solve problems is especially crucial for AI teams.
There must be understanding about the reasons behind the lack of diversity and the “bigger picture” of how powerful groups more easily perpetuate power structures already in place. Participants spoke of managers who did not belong to minoritized identities themselves but who took the time to learn in depth about differences in power and privilege in the tech ecosystem, appreciating the diverse perspectives that workers brought. These managers, while not perfect, tended to take advocating for their reports very seriously, particularly female reports who often went overlooked.
Intentionally diversifying leadership and managers will not automatically create a pipeline for diversity at the leadership level, nor will it automatically override institutional culture or policies that ignore DEI best practices.
3. DEI trainings must be specific in order to be effective and be more connected to the content of AI work
Almost all participants reported that their organizations mandated some form of DEI training for all staff. These ranged widely, from very general ones to very specific trainings that discussed cultural competency about more specific groups of people (e.g., participants reported that there were trainings on anti-Black racism). Participants discussed that the more specific trainings tended to be more impactful.
Organizations must invest in employees who see the importance of inclusive values in AI research and product design. Participants pointed to the importance of managers who had an ability to foster inclusive team values, which was not something that HR could mandate.
As several participants observed, DEI trainings will not uproot or counteract institutional stigmas against DEI. It would take sustained effort and deliberate alignment of values for an organization to emphasize DEI in its work.
4. Organizations must interrogate their values as practiced and fundamentally alter them to include the perspectives of people who are not White, cis, or male
Participants frequently reported that a misalignment of values was a primary reason for them leaving their organizations or wanting to leave their organizations. Participants in this sample discussed joining the AI field to create a positive impact while growing professionally. This led them to feeling disappointed when their organizations did not prioritize these goals (despite them being among their stated values).
Participants found it frustrating when organizations stated that they valued diversity and then failed to live up to this value with hiring, promotion, and day-to-day operations, ignoring the voices of minoritized individuals. If diversity is truly a value, organizations may have to investigate their systems of norms and expectations that are fundamentally male, Eurocentric, and do not make space for those from diverse backgrounds. They then must take additional steps to consider how such systems influence their work in AI.
Because achieving a fundamental re-alignment like this is a more comprehensive solution, it cannot satisfy the most immediate and urgent needs for reform. Short-term, organizations must work with DEI professionals to recognize how they are perpetuating potentially harmful norms of the dominant group and work to create policies that are more equitable. Longer term fixes may not, for instance, satisfy the immediate and urgent need for more diversity in leadership and teams in general.