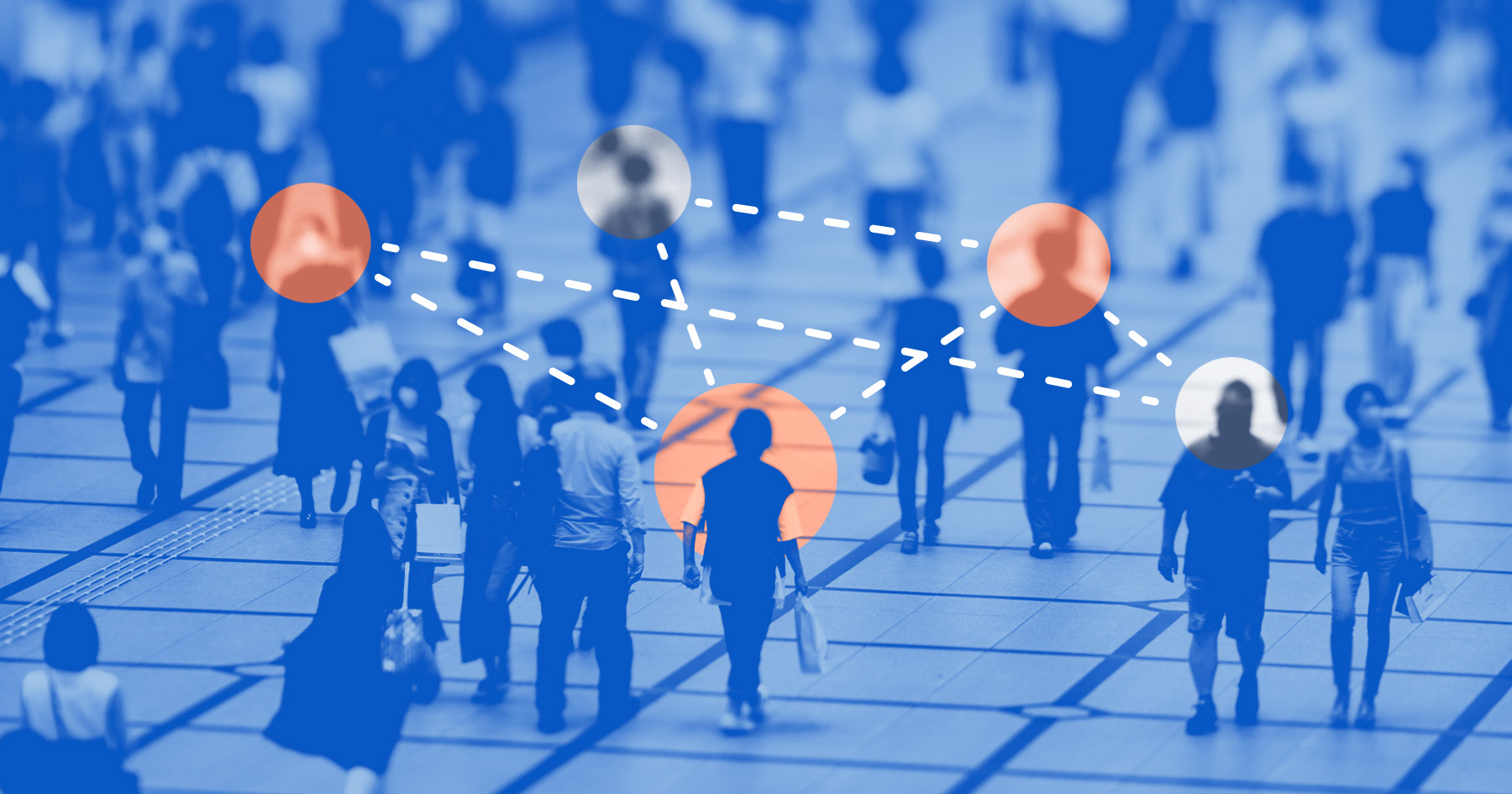
Making AI Inclusive: 4 Guiding Principles for Ethical Engagement
Introduction
While the concept of “human-centered design” is hardly new to the technology sector, recent years have seen growing efforts to build inclusive artificial intelligence (AI) and machine learning (ML) products. Broadly, inclusive AI/ML refers to algorithmic systems which are created with the active engagement of and input from people who are not on AI/ML development teams. This includes both end users of the systems and non-users who are impacted by the systems.✳“Impacted non-user” refers to people who are impacted by the deployment of an AI/ML system, but are not the direct user or customer of that system. For example, in the case of students in the United Kingdom in 2020 whose A-level grades were determined by an algorithm, the “user” of the algorithmic system is Ofqual, the official exam regulator in England, and the students are “impacted non-users.” To collect this input, practitioners are increasingly turning to engagement practices like user experience (UX) research and participatory design.
Amid rising awareness of structural inequalities in our society, embracing inclusive research and design principles helps signal a commitment to equitable practices. As many proponents have pointed out, it also makes for good business: Understanding the needs of a more diverse set of people expands the market for a given product or service. Once engaged, these people can then further improve an AI/ML product, identifying issues like bias in algorithmic systems.
Despite these benefits, however, there remain significant challenges to greater adoption of inclusive development in the AI/ML field. There are also important opportunities. For AI practitioners, AI ethics researchers, and others interested in learning more about responsible AI, this Partnership on AI (PAI) white paper provides guidance to help better understand and overcome the challenges related to engaging stakeholders in AI/ML development.
Ambiguities around the meaning and goals of “inclusion” present one of the central challenges to AI/ML inclusion efforts. To make the changes needed for a more inclusive AI that centers equity, the field must first find agreement on foundational premises regarding inclusion. Recognizing this, this white paper provides four guiding principles for ethical engagement grounded in best practices:
- All participation is a form of labor that should be recognized
- Stakeholder engagement must address inherent power asymmetries
- Inclusion and participation can be integrated across all stages of the development lifecycle
- Inclusion and participation must be integrated to the application of other responsible AI principles
To realize ethical participatory engagement in practice, this white paper also offers three recommendations aligned with these principles for building inclusive AI:
- Allocate time and resources to promote inclusive development
- Adopt inclusive strategies before development begins
- Train towards an integrated understanding of ethics
This white paper’s insights are derived from the research study “Towards An Inclusive AI: Challenges and Opportunities for Public Engagement in AI Development.” That study drew upon discussions with industry experts, a multidisciplinary review of existing research on stakeholder and public engagement, and nearly 70 interviews with AI practitioners and researchers, as well as data scientists, UX researchers, and technologists working on AI and ML projects, over a third of whom were based in areas outside of the US, EU, UK, or Canada. Supplemental interviews with social equity and Diversity, Equity, and Inclusion (DEI) advocates contributed to the development of recommendations for individual practitioners, business team leaders, and the field of AI and ML more broadly.
This white paper does not provide a step-by-step guide for implementing specific participatory practices. It is intended to renew discussions on how to integrate a wider range of insights and experiences into AI/ML technologies, including those of both users and the people impacted (either directly or indirectly) by these technologies. Such conversations — between individuals, inside teams, and within organizations — must be had to spur the changes needed to develop truly inclusive AI.