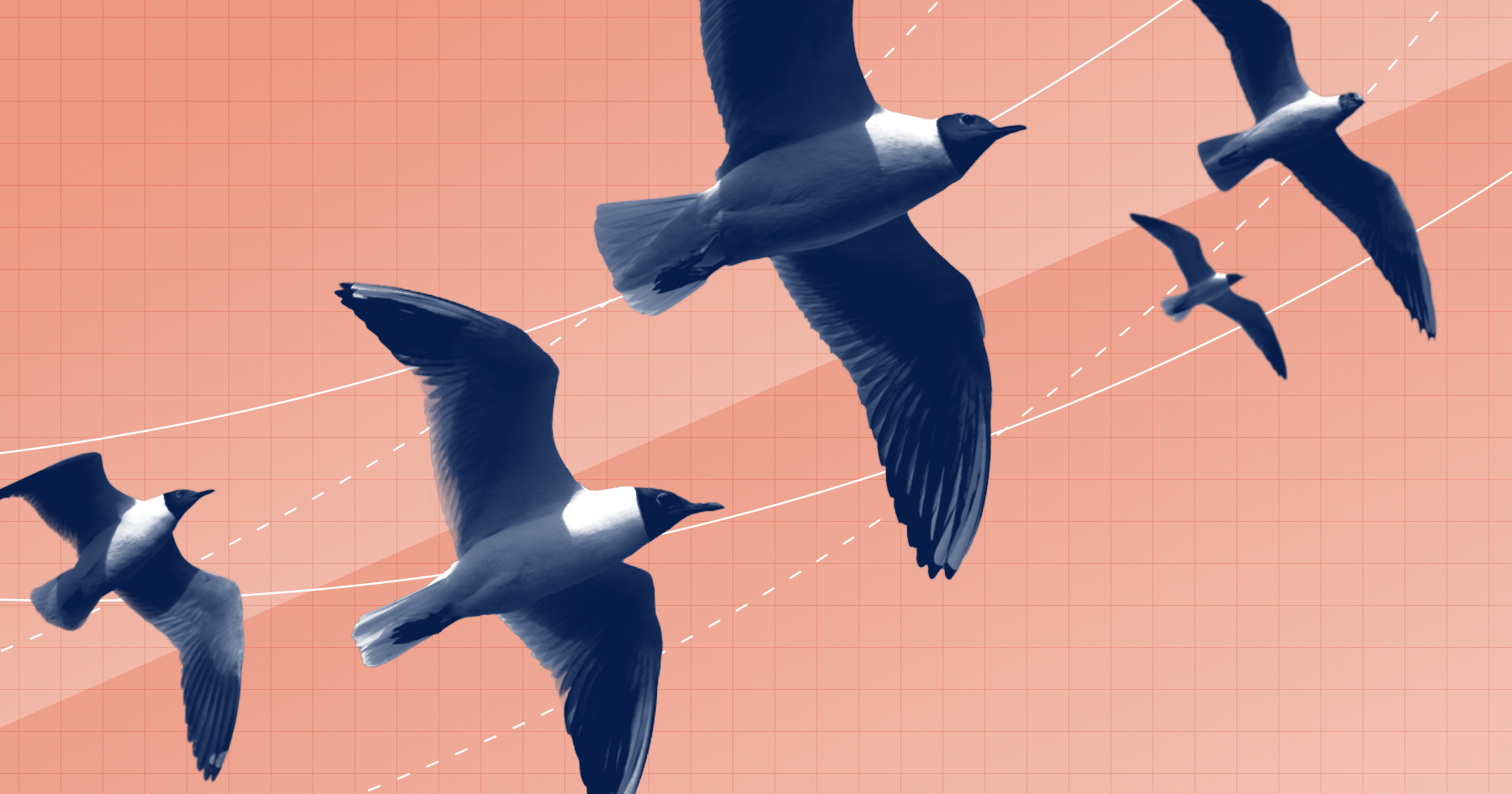
Guidelines for AI and Shared Prosperity
Step 2: Apply the Job Impact Assessment Tool
Step 2: Apply the Job Impact Assessment Tool
Use the high-level Job Impact Assessment Tool to analyze a given AI system:
Go over the full list of signals of opportunity and risk
Analyze the distribution of potential benefits and harms
Repeat this process for upstream and downstream markets
Instructions for Performing a Job Impact Assessment
Instructions for Performing a Job Impact Assessment
For each signal, if you estimated the likelihood of the respective opportunity or risk materializing as a result of the introduction of the AI system into the economy to be anything but “zero,” please note the respective signal as “present.”
Certainty in likelihood estimation is not a prerequisite for this high-level assessment and is assumed to be absent in most cases. When in doubt, note the signal as “present.”
Policymakers, workers and their representatives can use the results of the high-level Jobs Impact Assessment to inform their decisions, actions, and agendas as outlined in the Suggested Uses section under Step 3 of the Shared Prosperity Guidelines. We look forward to collecting feedback on the Guidelines and curating use examples in partnership with interested stakeholders. To get involved, please get in touch.
Signals of Opportunity to Advance Shared Prosperity
Signals of Opportunity to Advance Shared Prosperity
If one or more of the statements below apply to the AI system being assessed, this indicates a possibility of a positive impact on shared prosperity-relevant outcomes.
An opportunity signal (OS) is present if an AI system may:
How significant and widely distributed consumer benefits should be to justify job losses is a political question,✱For example, in 2011, the US government imposed tariffs to prevent job losses in the tire industry. Economic analysis later showed that the tariffs cost American consumers around $0.9 million per job saved: https://www.piie.com/publications/policy-briefs/us-tire-tariffs-saving-few-jobs-high-cost. It seems implausible that such large consumer costs are worthwhile, relative to the job gains. but quantifying consumer gains per job lost would help sharpen up any debate about the value of an AI innovation.✱In this paper, Brynjolfsson et al. estimate the value of many free digital goods and services: https://www.pnas.org/doi/10.1073/pnas.1815663116. They do so by proposing a new metric called GDP-B, which quantifies their benefits rather than costs, and then estimating consumers’ willingness-to-pay for free digital goods and services in terms of GDP-B.Brynjolfsson, E., Collis, A., Diewert, W.E., Eggers, F., and Fox, K.J. (2019). GDP-B: Accounting for the value of new and free goods in the digital economy (No. w25695). National Bureau of Economic Research. As stated in “Key Principles for Using the Guidelines,” independently of the magnitude and distribution of anticipated benefits, appropriate mitigation strategies should be developed in response to the risk of job losses or wage decreases.
Will the AI system boost productivity of workers, in particular those in lower-paid jobs, without increasing strain? By a worker’s productivity, we mean a worker’s output per hour. A more productive worker is more valuable to their employer and (all other conditions remaining the same) is expected to be paid more.✱As emphasized in Key Principles for Using the Guidelines, signals of opportunity are not guarantees: It is possible that the introduction of a new technology into the workplace boosts workers’ productivity but does not lead to wage growth because, in practice, workers’ productivity is only one of the factors determining their wage. Other factors include how competitive the market is and how much bargaining power workers have. In fact, a large number of countries have been experiencing productivity-wage decoupling in recent decades (see, for example: https://www.oecd.org/economy/decoupling-of-wages-from-productivity/). This points to a diminishing role of productivity in determining wages, but it remains non-zero and hence has to be accounted for by the Guidelines. Therefore, if an AI system comes with a promise of a productivity boost that is a positive signal. Besides, productivity growth is often the prerequisite for the creation of consumer benefits discussed in OS1.However, three important caveats should be noted here.
Caveat 1: Productivity boosts can deepen inequality
It is quite rare for a technology to equally boost productivity for everyone involved in the production of a certain good, more often it helps workers in certain skill groups more than others. If it is helping workers in lower-paying jobs relatively more, the effect could be inequality-reducing. Otherwise, it may be inequality-deepening. Please document the distribution of the productivity increase across the labor force when assessing the presence of this opportunity signal.
Caveat 2: Productivity boosts can displace workers
Even if productivity of all workers involved in the production of a certain good is boosted equally by an AI system, fewer of them might find themselves employed in the production of that good once the AI system is in place. This is because fewer (newly more productive) worker-hours✱The impact of a productivity-enhancing technology can manifest itself as a reduction of the size of the workforce, or a reduction in hours worked by the same-size labor force. Either option can negatively impact shared prosperity. are now needed to create the same volume of output. For production of the good in question to require more human labor after AI deployment, two conditions must be met:
- Productivity gains of the firm introducing AI need to be shared with its clients (such as consumers, businesses, or governments) in the form of lower-priced or higher-quality products — something which is less likely to happen in a monopolistic environment
- Clients should be willing to buy sufficiently more of that lower-priced or higher-quality product
If the first condition is met but the second is not, the introduction of the AI system in question might still be, on balance, labor-demand boosting if it induces a “productivity effect” in the broader economy. When productivity gains and corresponding consumer benefits are sufficiently large, consumers will experience a real income boost generating new labor demand in the production of complementary goods. That new labor demand might be sufficient to compensate for the original loss of employment due to an introduction of an AI system. Issues arise when the productivity gains are too small like in the case of “so-so” technologiesAcemoglu, D., and Restrepo, P. (2019). Automation and new tasks: How technology displaces and reinstates labor. Journal of Economic Perspectives, 33(2), 3-30. or are not shared with consumers. If that is the case, please document OS2 as “not present” when performing the Job Impact Assessment.
Caveat 3: Productivity boosts can significantly hamper job quality
Introduction of an AI system can lead to productivity enhancement through various routes: by allowing workers to produce more output per hour of work at the same level of effort or by allowing management to induce a higher level of effort from workers. If productivity boosts are expected to be achieved solely or mainly through increasing work intensity, please document OS2 as “not present” when performing the Job Impact Assessment.
Lastly, frontline workersBell, S. A. (2022). AI and Job Quality: Insights from Frontline Workers. https://papers.ssrn.com/sol3/papers.cfm?abstract_id=4337611 reported appreciation for AI systems that boosted their productivity by assisting them with core tasks. Conversely, technologies that boosted productivity by automating workers’ core tasks were associated with a reduction in job satisfaction.Valentine, M., and Hinds, R. (2022). How Algorithms Change Occupational Expertise by Prompting Explicit Articulation and Testing of Experts’ Theories. https://tinyurl.com/pxyr8ev3 Hence, pursuit of productivity increases through technologies that eliminate non-core tasks is preferred over paths that involve eliminating core tasks. Examples of technologies that assist workers on their core tasks include:
- Training and coaching tools
- Algorithmic decision support systems that give users additional information, analytics, or recommendations without prescribing or requiring decisions
Will the AI system create new tasks for humans or move unpaid tasks into paid work? Technological innovations have a great potential for benefit when they create new formal sector jobs, tasks, or markets that did not exist before. Consider, for example, the rise of social media influencers and content creators. These types of jobs were not possible before the rise of contemporary media and recommendation technologies. It has been estimated that, in 2018, more than 60 percent of employees were employed in occupations that did not exist in 1940.Autor, D. (2022). The labor market impacts of technological change: From unbridled enthusiasm to qualified optimism to vast uncertainty (No. w30074). National Bureau of Economic Research.
Caveat 1: Someone’s unpaid tasks can be someone else’s full-time job
It is important to keep in mind that technologies seemingly moving unpaid tasks into paid ones might, upon closer inspection, be producing an unintended (or deliberately unadvertised) effect of shifting tasks between paid jobs — often accompanied by a job quality downgrade. For example, a technology that allows people to hire someone to do their grocery shopping might convert their unpaid task into someone else’s paid one, but also reduce the demand for full-time domestic help workers, increasing precarity in the labor market.
Caveat 2: New tasks often go unacknowledged and unpaid
Sometimes the introduction of an AI system adds unacknowledged and uncompensated tasks to the scope of workers. For example, the labor of smoothing the effects of machine malfunction remains under the radar in many contexts,Mateescu, A., and Elish, M. (2019). AI in context: the labor of integrating new technologies. creating significant unacknowledged burdens on workers who end up responsible for correcting machine’s errors (without being adequately positioned to do that).Elish, M. C. (2019). Moral crumple zones: Cautionary tales in human-robot interaction (pre-print). Engaging Science, Technology, and Society (pre-print).
When performing the Job Impact Assessment, please explicitly document the applicability of these two caveats associated with OS3 for the AI system being assessed and its deployment context.
Consequently, lower-income countries would greatly benefit from access to technologies that would allow them to stay competitive by leveraging their abundant labor resources and creating gainful jobs that do not require high levels of educational attainment.
When assessing the presence of this signal, please also document if and how the relative abundance of capital and labor of various skill types is expected to change over time.
Will the AI system broaden access to the labor market? AI systems that allow communities with limited or no access to formal employment to get access to gainful formal sector jobs are highly desirable from the perspective of broadly shared prosperity. Examples include AI systems that:
- Assist the disabled
- Make it easier to combine work and caregiving responsibilities
- Enable work in languages the worker does not have a fluent command of
Please note that worker benefits are included in workers’ share of an organization’s revenue. For example, consider an organization that adopts a productivity-enhancing AI system which allows it to produce the same or greater amount of output with fewer hours of work needed from human workers. That organization can decide to retain the same size of the workforce and share productivity gains with it (for example, in the form of higher wages, longer paid time off, or shorter work week at constant weekly pay), keeping the workers’ share of revenue constant or growing. That would be a prime example of using AI to advance shared prosperity.
Lastly, if an organization was able to generate windfall gains from AI development or usage and is committed to sharing the gains not only with workers it directly employs but the rest of the world’s population as well, that can be a great example of using AI to advance shared prosperity. While some have proposed this,O’Keefe, C., Cihon, P., Garfinkel, B., Flynn, C., Leung, J., and Dafoe, A. (2020, February). The windfall clause: Distributing the benefits of AI for the common good. In Proceedings of the AAAI/ACM Conference on AI, Ethics, and Society (pp. 327-331). more research is needed to design mechanisms for making sure windfall gains are distributed equitably and organizations can be expected to reliably honor their commitment to distribute their gains.
Were workers who will ultimately use or be affected by the AI system (or their representatives) included and given agency in every stage of the system’s development? Workers are subject matter experts in their own tasks and roles, and can illuminate opportunities and challenges for new technologies that are unlikely to be seen by those with less familiarity with the specifics of the work. The wisdom of workers who use or are most affected by AI systems introduced throughout development can smooth many rough edges that other contributors might only discover after systems are in the market and implemented. Where relevant worker representatives exist, they should be brought into the development process to represent collective worker interests from start to finish.Fully offering affected workers agency in the development process requires taking the time to understand their vantage points, and equip them or their representatives with enough knowledge about the proposed technology to meaningfully participate. They also must be afforded sufficient decision-making power to steer projects and, if necessary, end them in instances where unacceptable harms cannot be removed or mitigated. This also necessitates protecting their ability to offer suggestions freely without fear of repercussions. Without taking these steps, participatory processes can still lead to suboptimal outcomes — and possibly create additional harms through covering problems with a veneer of worker credibility.
Caveat 1: Systems can improve one aspect of job quality while harming another
For example, many AI technologies positioned as safety enhancements are in reality invasive surveillance technologies. Though safety improvements may occur, harms to human rights, stress rates, privacy, job autonomy, job intensity, and other aspects of job quality may occur as well. Other AI systems purport to improve job quality by automating tasks workers dislike (see RS1 for more detail on the risks of task elimination).
When a system enhances one aspect of job quality while endangering another, this signal can still be counted as “present,” but the need to consider the rest of the opportunity and risk signals is particularly important.
Caveat 2: AI systems are sometimes deployed to redress job quality harms created by other AI systems
For example, some companies have introduced AI safety technologies to correct harms resulting from the prior introduction of an AI performance target-setting system that encouraged dangerous overwork.Scherer, M., and Brown, L. X. (2021). Warning: Bossware May Be Hazardous to Your Health. Center for Democracy and Technology. https://cdt.org/wp-content/uploads/2021/07/2021-07-29-Warning-Bossware-May-Be-Hazardous-To-Your-Health-Final.pdf
When this is the case, the introduction of the new AI system to redress the harms of the old does not count for this signal and should be marked as “not present.”
Instead of introducing new AI systems with their own attendant risks, the harms from the existing systems should be addressed in line with the Responsible Practices provided by the Guidelines for AI-using organizations and additional case-specific mitigations.
Signals of Risk to Shared Prosperity
Signals of Risk to Shared Prosperity
For-profit companies might feel pressure from investors to cut their labor costs no matter the societal price. We encourage investors and governments to join civil society in an effort to incentivize responsible business behavior with regards to shared prosperity and labor market impact.
Some practices or outcomes included in this section are illegal in some jurisdictions, and as such are already addressed in those locations. We include them here due to their legality in other jurisdictions.
Some of the signals of risk to shared prosperity described in the Guidelines are actively sought by companies as profit-making opportunities. The Guidelines do not suggest that companies should stop seeking profits, just that they should do so responsibly.
Profit-generating activities do not necessarily have to harm workers and communities, but some of them do. The presence of signals of risk indicates the potential to impose undue costs on society, which require mitigation strategies.
A risk signal (RS) is present if an AI system may:
Task-related Risks
However, if an AI system is primarily geared towards eliminating core paid tasks without much being expected in terms of increased job quality or broadly shared benefits, nor in terms of new tasks for humans being created in parallel, then it warrants further attention as posing a risk to shared prosperity. The introduction of such a system will likely lower the demand for human labor, and thus wage or employment levels for affected workers.Acemoglu, D., and Restrepo, P. (2022). Tasks, automation, and the rise in US wage inequality. Econometrica, 90(5), 1973-2016. Automation of core tasks can also be experienced by workers as directly undermining their job satisfaction since workers’ core responsibilities are closely tied to their sense of pride and accomplishment in their jobs. For workers who see their jobs as an important part of their identity, core tasks are a major aspect of how they see themselves in the world.Valentine, M., and Hinds, R. (2022). How Algorithms Change Occupational Expertise by Prompting Explicit Articulation and Testing of Experts’ Theories. https://tinyurl.com/pxyr8ev3 Automation of core tasks can also lower the skill requirements of a job and reduce the formation of skills needed to advance to the next level.Nurski, L., and Hoffmann, M. (2022). The Impact of Artificial Intelligence on the Nature and Quality of Jobs. Working Paper. Bruegel. https://tinyurl.com/jxayzdcz
Please note that to evaluate the share of a given job’s tasks being eliminated, those tasks should be weighted by their importance for the production of the final output. We consider task elimination above 10% significant enough to warrant attention.
Paid tasks can also be converted into unpaid when new technology enables them to be performed by customers. Examples of that are self-checkout kiosks or automated customer support.Pritchett, L. (2020). The future of jobs is facing one, maybe two, of the biggest price distortions ever. Middle East Development Journal, 12(1), 131-156.
Importantly, AI-induced reallocation of tasks to jobs with lower specialized skills requirements may be positive but is still a risk signal warranting further attention, because lowering specialized skill requirements can lower not only the barriers to entry to the occupation, but also prevailing wages.
Market-related Risks
In addition to jobs disappearing as the direct effect of labor-saving technology being introduced in a region, please note that this effect can also be an indirect result of labor-saving technology initially introduced in a completely different region or country. Due to excessive immigration barriers, AI developers based in high-income countries face massively inflated incentives to create labor-saving technologies far in excess of what would be socially optimal given the world’s overall level of labor supply/demand for jobs.Pritchett, L. (2020). The future of jobs is facing one, maybe two, of the biggest price distortions ever. Middle East Development Journal, 12(1), 131-156. Once that technology is developed in the high-income countries it gets deployed all over the world, including countries facing a dire need of formal sector jobs.Pritchett, L. (2023). Choose People. LaMP Forum. https://lampforum.org/2023/03/02/choose-people/
- It increases the risk of job cuts by competing firms
- It makes it less likely that the winning firm shares efficiency gains with workers in the form of better wages/benefits or with consumers in the form of lower prices/higher-quality products
Therefore, in a monopolistic market, any benefits brought on by AI are likely to be shared by few, while the harms might still be widely distributed. Similarly, job impacts that might occur in upstream or downstream industries due to an AI-induced increase in market concentration need to be accounted for as well.
Sourcing-related Risks
- Inconsistent and unpredictable compensation for their work
- Unfairly rejected and therefore unpaid labeling tasks
- Long, ad-hoc working hours
- Lack of means to contest or get an explanation for the decisions affecting their take-home pay and ratings
Lack of transparency around data enrichment labor sourcing practices in the AI industry exacerbate this issue.
- Images created by artists and photographers that are used to train generative AI systems
- Keystrokes and audio recordings of human customer service agents used to create automated customer service routines
- Records of actions taken by human drivers used to train autonomous driving systems
Worker Abuse-related Risks
- Emotional well-being through increased stress
- Occupational safety and health through sleep deprivation/unpredictability and the physical effects of stress
- Financial well-being through missed shifts and increased need for more expensive transit (for example, ride-hailing services at times when public transit isn’t frequent or safe).
Recent AI technology designed to lower labor costs by reducing the number of people working during predicted “slow” times has disrupted schedule predictability, with workers receiving minimal notice about hours that have been eliminated from or added to their schedules.
- Increasing stress and anxiety
- Harming their privacy
- Causing them to feel a lack of trust from their employer
- Undermining their sense of autonomy on the job
- Lowering engagement and job satisfaction
- Chilling worker organizing, undermining worker voice.Moore, P.V. (2017). The quantified self in precarity: Work, technology and what counts. Routledge.Scherer, M., and Brown, L. X. (2021). Warning: Bossware May Be Hazardous to Your Health. Center for Democracy and Technology. https://cdt.org/wp-content/uploads/2021/07/2021-07-29-Warning-Bossware-May-Be-Hazardous-To-Your-Health-Final.pdf.
While monitoring systems can have legitimate uses (such as enhancing worker safety), even good systems can be abused, particularly in environments with low worker agency or an absence of regulations, monitoring, and enforcement of worker protections.Brand, J., Dencik, L. and Murphy, S. (2023). The Datafied Workplace and Trade Unions in the UK. Data Justice Lab. https://datajusticeproject.net/wp-content/uploads/sites/30/2023/04/Unions-Report_final.pdf.