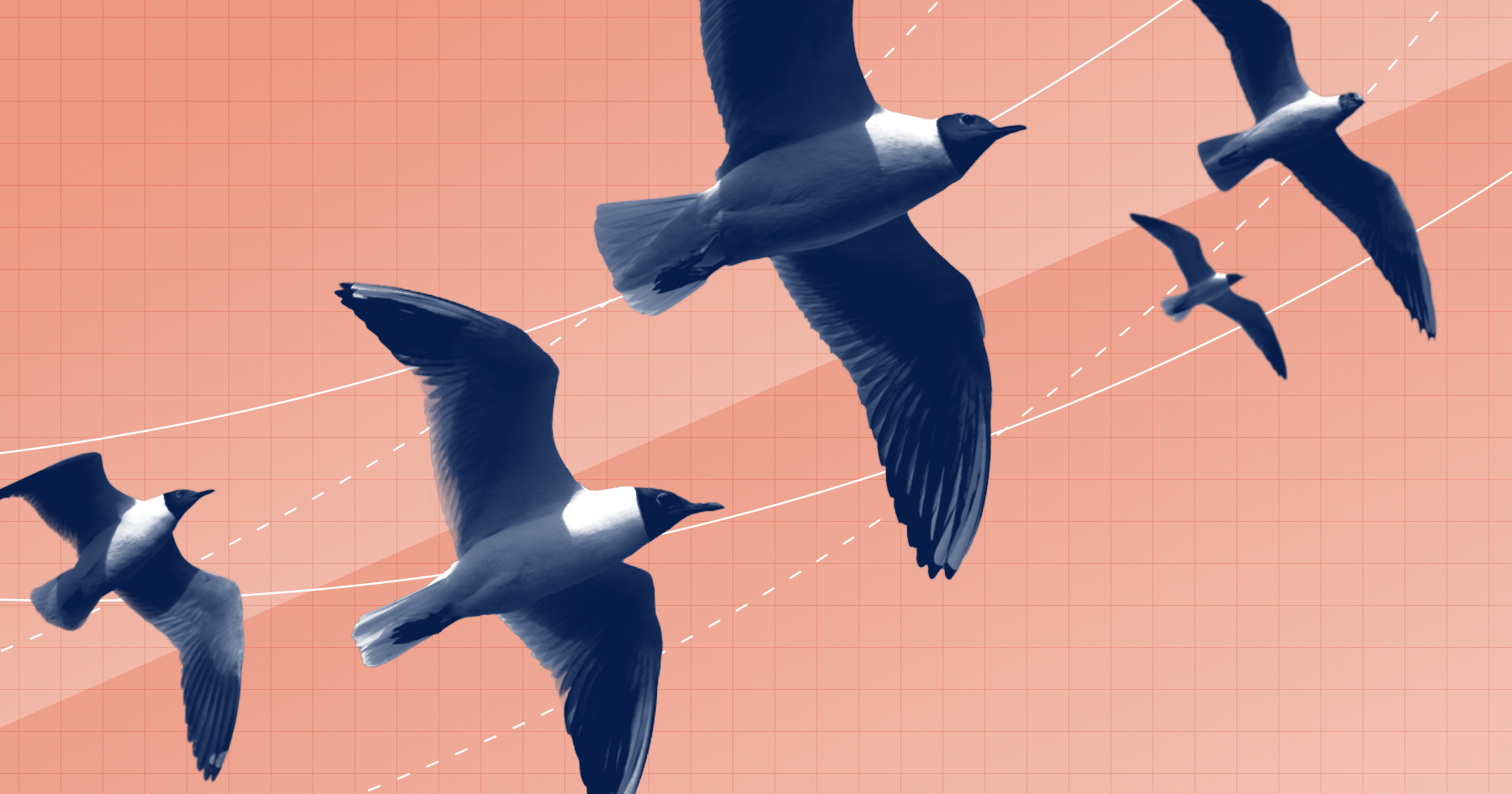
Guidelines for AI and Shared Prosperity
For AI-Creating Organizations
Responsible Practices for AI-Creating Organizations (RPC)
After performing the High-Level Job Impact Assessment, consult our recommendations to help minimize the risks and maximize the opportunities to advance shared prosperity with AI.
Use of workplace AI is still in early stages, and as a result information about what should be considered best practices for fostering shared prosperity is still preliminary. Below is a list for AI-creating organizations of starter sets of practices aligned with increasing the likelihood of benefits to shared prosperity and decreasing the likelihood of harms to it. The list is drawn from early empirical research in the field, historical analogues for transformative workplace technologies, and theoretical frameworks yet to be applied in practice. For ease of use, the lists of Responsible Practices are organized by the earliest AI system lifecycle stage where the practice can be applied.
At an organizational level
Multiple AI-creating organizations aspire (according to their mission statements and responsible AI principles) to develop AI that benefits everyone. Very few of them, however, currently publicly acknowledge the scale of labor market disruptions their AI systems might bring about or make efforts to help communities that stand to be affected have a say in the decisions determining the path, depth, and distribution of labor market disruptions. At the same time, AI-creating organizations are often best positioned to anticipate labor market risks well in advance of those becoming apparent to other stakeholders, thus making risk disclosures by AI-creating organizations a valuable asset for governments and societies.
The public commitment to disclose severe risks✱PAI’s Shared Prosperity Guidelines use UNGP’s definition of severity: an impact (potential or actual) can be severe “by virtue of one or more of the following characteristics: its scale, scope or irremediability. Scale means the gravity of the impact on the human right(s). Scope means the number of individuals that are or could be affected. Irremediability means the ease or otherwise with which those impacted could be restored to their prior enjoyment of the right(s).” https://www.ungpreporting.org/glossary/severe-human-rights-impact/ should specify the severity threshold considered by the organizations to warrant disclosure, as well as explain how the threshold level of severity was chosen and what external stakeholders were consulted in that decision.
Alternatively, an organization can choose to set a threshold in terms of an AI system’s anticipated capabilities and disclose all risk signals which are present for those systems. For example, if the expected return on investment from the deployment of an AI system is a multiple greater than 10, or more than one million US dollars were spent on training compute and data enrichment, its corresponding risks would be subject to disclosure.✱These thresholds are used for illustrative purposes only: AI creating organizations should set appropriate thresholds and explain how they were arrived at. Thresholds need to be reviewed and possibly revised regularly as the technology advances.
During the full AI lifecycle
Run opportunity and risk analyses early and often in the AI research and product development process, using the data available at each stage. Update as more data becomes available (for example, as product-market fit becomes clearer or features are built out enough for broader worker testing and feedback). Whenever applicable, we suggest using AI system design and deployment choices to maximize the presence of signals of opportunity and minimize the presence of signals of risk.
Always solicit the input of workers that stand to be affected — both incumbents as well as potential new entrants — and a multi-disciplinary set of third-party experts when assessing the presence of opportunity and risk signals. Make sure to compensate external contributors for their participation in the assessment of the AI system.
Please note that the analysis of opportunity and risk signals suggested here is different from red team analysis suggested in RPC13. The former identifies risks and opportunities created by an AI system working perfectly as intended. The latter identifies possible harms if the AI system in question malfunctions or is misused.
In alignment with UN Guiding Principles for Business and Human Rights, a mitigation strategy should be developed for each risk identified, prioritizing the risks primarily by severity of potential impact and secondarily by its likelihood. Severity and likelihood of potential impact are determined on a case-by-case basis.✱An algorithm described here is very useful for determining the severity of potential quantitative impacts (such as impacts on wages and employment), especially in cases with limited uncertainty around the future uses of the AI system being assessed: https://www.brookings.edu/research/how-innovation-affects-labor-markets-an-impact-assessment/
Mitigation strategies can range from eliminating the risk or reducing the severity of potential impact to ensuring access to remedy or compensation for affected groups. If effective mitigation strategies for a given risk are not available, this should be considered a strong argument in favor of meaningful changes in the development plans of an AI system, especially if it is expected to affect vulnerable groups.
Engaging adequately compensated external stakeholders in the development of mitigation strategies is critical to ensure important considerations are not being missed. It is especially critical to engage with representatives of communities that stand to be affected.
Key requirements for the responsible sourcing of data enrichment services (such as, data annotation and real-time human verification of algorithmic predictions) include:
- Always paying data enrichment workers above the local living wage
- Providing clear, tested instructions for data enrichment tasks
- Equipping workers with simple and effective mechanisms for reporting issues, asking questions, and providing feedback on the instructions or task design
In collaboration with our Partners, PAI has developed a library of practitioner resources for responsible data enrichment sourcing.
During system origination and development
Workers who will use or be affected by AI hold unique perspectives on important needs and opportunities in their roles. They also possess particular insight into how AI systems could create harm in their workplaces. To ensure AI systems foster shared prosperity, these workers should be given agency in the AI development process from start to finish.
This work does not stop at giving workers a seat at the table throughout the development process. Workers must be properly equipped with knowledge of product functions, capabilities, and limitations so they can draw meaningful connections to their role-based knowledge. Additionally, care must be taken to create a shared vocabulary on the team, so that technical terms or jargon do not unintentionally obscure or mislead. Workers must also be given genuine decision-making power in the process, allowing them to shape product functions and features, and be taken seriously on the need to end a project if they identify unacceptable harms that cannot be resolved.
AI systems welcomed by workers largely fall into three overarching categories:
- Systems that directly improve some element of job quality
- Systems that assist workers to achieve higher performance on their core tasks
- Systems that eliminate undesirable non-core tasks (See OS3, RS1, and RS2 for additional detail)
Starting with one of these objectives in mind and creating robust participation mechanisms for workers throughout the design and implementation process is likely to result in win-win-wins for AI creators, employers who implement AI, and the workers who use or are affected by them.
A given AI system complements a certain group of workers if the demand for labor of that group of workers can be reasonably expected to go up when the price of the use of that AI system goes down. A given AI system is a substitute for a certain group of workers if the demand for labor of that group of workers is likely to fall when the price of the use of that AI system goes down.
Note that the terms “labor-augmenting” technology and “labor-complimentary” technology are often erroneously used interchangeably. “Labor-augmenting technology” is increasingly being used as a loose marketing term which frames workplace surveillance technology as worker-assistive.Klinova, K. (2022) Governing AI to Advance Shared Prosperity. In Justin B. Bullock et al. (Eds.), The Oxford Handbook of AI Governance. Oxford Handbooks.
Getting direct input from workers is very helpful for differentiating genuinely complementary technology from the substituting kind. Please also see the discussion of the distinction between core and non-core tasks and the acceptable automation thresholds in RS1.
In general, AI systems frequently reproduce or deepen discriminatory patterns in society, including ones related to race, class, age, and disability. Specific workplace systems have shown a propensity for the same. Careful work is needed to ensure any AI systems affecting workers or the economy do not create discriminatory results.
Before selling or deploying the system
The field of explainable AI has advanced considerably in recent years, but workers remain an underrepresented audience for AI explanations.Park, H., Ahn, D., Hosanagar, K., and Lee, J. (2021, May). Human-AI interaction in human resource management: Understanding why employees resist algorithmic evaluation at workplaces and how to mitigate burdens. In Proceedings of the 2021 CHI Conference on Human Factors in Computing Systems (pp. 1-15). Providing workers explanations of workplace AI systems tailored to the particulars of their roles and job goals enables them to understand the tools’ strengths and weaknesses. When paired with workers’ existing subject matter expertise in their own roles, this knowledge equips workers to most effectively attain the upsides and minimize the downsides of AI systems, meaning AI systems can enhance their overall job quality across the different dimensions of well-being.
Privacy and ownership over data generated by one’s activities are increasingly rights recognized inside and outside the workplace. Respect for these rights requires fully informing workers about the data collected on them and inferences made, how they are used and why, as well as offering them the ability to opt out of collection and use.Bernhardt, A., Suleiman, R., and Kresge, L. (2021). Data and algorithms at work: the case for worker technology rights. https://laborcenter.berkeley.edu/wp-content/uploads/2021/11/Data-and-Algorithms-at-Work.pdf. Workers should also be given the opportunity to individually or collectively forbid the sales of datasets that include their personal information or personally identifiable information. In particular, system design should follow the data minimization principle: collect only the necessary data, for the necessary purpose, and hold it only for the necessary amount of time. Design should also enable workers to know about, correct, or delete inferences about them. Particular care must be taken in workplaces, as the power imbalance between employer and employee undermines workers’ ability to freely consent to data collection and use compared to other, less coercive contexts.Colclough, C.J. (2022). Righting the Wrong: Putting Workers’ Data Rights Firmly on the Table. https://tinyurl.com/26ycnpv2
AI systems have been built to hire workers, manage them, assess their performance, and promote or fire them. AI is also being used to assist workers with their tasks, coach them, and complete tasks previously assigned to them. In each of these decisions allocated to AI, the technologies have accuracy as well as comprehensiveness issues. AI systems lack the human capacity to bring in additional context relevant to the issue at hand. As a result, humans are needed to validate, refine, or override AI outputs. In the case of task completion, an absence of human involvement can create harms to physical, intellectual, or emotional well-being. In AI’s use in employment decisions, it can result in unjustified hiring or firing decisions. Simply placing a human “in the loop” is insufficient to overcome algorithmic bias: demonstrated patterns of deference to the judgment of algorithmic systems. Care must be taken to appropriately position the strengths and weaknesses of AI systems and empower humans with final decision-making power.Pasquale, F. (2020). New Laws of Robotics. Harvard University Press.
AI systems are less likely to cause harm in environments with:
- High levels of legal protection, monitoring, and enforcement for workers’ rights (such as those related to health and safety or freedom to organize)
- High levels of worker voice and negotiating ability (due to strong protections for worker voice or high demand for workers’ comparatively scarce skills), especially those where workers have meaningful input into decisions regarding the introduction of new technologies
These factors encourage worker-centric AI design. Workers in such environments also possess a higher ability to limit harms from AI systems (such as changing elements of an implementation or rejecting the use of the technology as needed), including harms outside direct legal protections. This should not, however, be treated as a failsafe for harmful technologies, particularly when AI systems can easily be adopted in environments where they were not originally intended.Rodrik, D. (2022). 4 Prospects for global economic convergence under new technologies. An inclusive future? Technology, new dynamics, and policy challenges, 65. In environments where workers lack legal protection and/or decision-making power, it is especially important to scrutinize uses and potential impacts, building in additional mitigations to compensate for the absence of these worker safeguards. Contractual or licensing provisions regarding terms of use, rigorous customer vetting, and geofencing are some of the many steps AI-creating organizations can take to follow this practice. Care should be taken to adopt fine-grained mitigation strategies where possible such that workers and economies can reap the gains of neutral or beneficial uses.
The preceding points have focused on AI systems working as designed and intended. Responsible development also requires comprehensive “red teaming” of AI systems to identify vulnerabilities and the potential for misuse or abuse. Adversarial ML is increasingly a part of standard security practice. Additionally, the development team, workers in relevant roles, and external experts should test the system for misuse and abusive implementation.
The power and responsibility to share productivity gains from AI system implementation lies mostly with AI-using organizations. The role of AI-creating organizations is to make sure the functionality of an AI system does not fundamentally undermine opportunities for workers to share in productivity gains, which would be the case if an AI system de-skills jobs and makes workers more likely to be viewed as fungible or automates a significant share of workers’ core tasks.
The benefit to workers and society from following these practices can be meaningfully undermined if organizations deploying or using the AI system do not do their part to advance shared prosperity. We encourage developers to make adherence to the Guidelines’ Responsible Practices a contractual obligation during the selling or licensing of the AI system for deployment or use by other organizations.
Shared Prosperity Guidelines Home
Get Involved
Partnership on AI needs your help to refine, test, and drive adoption of the Shared Prosperity Guidelines.
Fill out the form below to share your feedback on the Guidelines, ask about collaboration opportunities, and receive updates about events and other future work by the AI and Shared Prosperity Initiative.