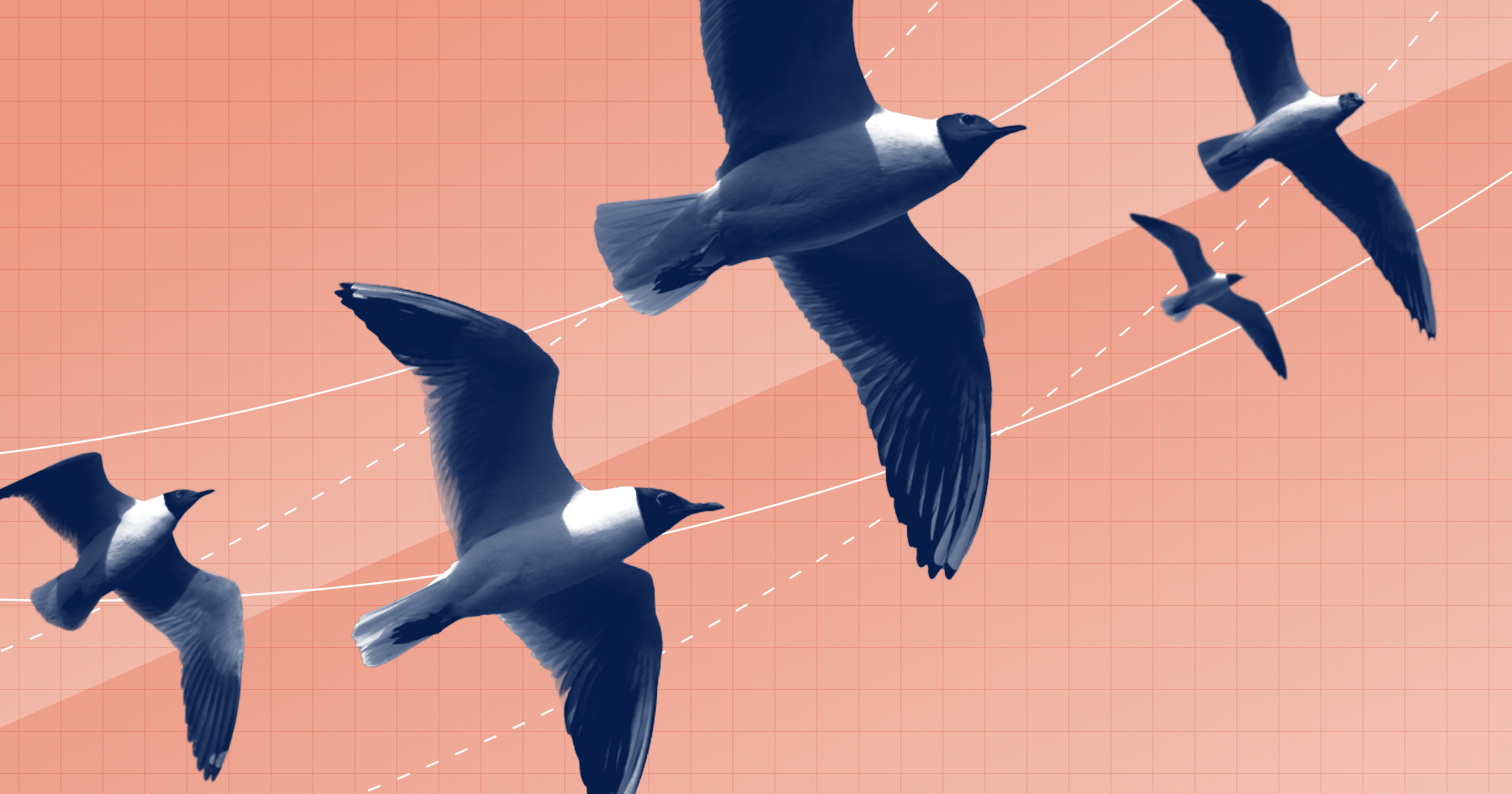
Guidelines for AI and Shared Prosperity
For AI-Using Organizations
Responsible Practices for AI-Using Organizations (RPU)
After performing the High-Level Job Impact Assessment, consult our recommendations to help minimize the risks and maximize the opportunities to advance shared prosperity with AI.
Use of workplace AI is still in early stages, and as a result information about what should be considered best practices for fostering shared prosperity is still preliminary. Below is a list for AI-using organizations of starter sets of practices aligned with increasing the likelihood of benefits to shared prosperity and decreasing the likelihood of harms to it. The list is drawn from early empirical research in the field, historical analogues for transformative workplace technologies, and theoretical frameworks yet to be applied in practice. For ease of use, the lists of Responsible Practices are organized by the earliest AI system lifecycle stage where the practice can be applied.
At an organizational level
Labor practices and impacts are increasingly a part of suggested, proposed, or required non-financial disclosures. These disclosures include practices affecting human rights, management of human capital, and other social and employee issues. Regulatory authorities have suggested, proposed, or required these disclosures as material to investor decision-making, as well as for the benefit of the broader society. We recommend that AI-using organizations identify, disclose, and mitigate the risks of severe labor market impacts for the same rationales, as well as to provide both prospective and existing workers with the information they need to make informed decisions about their own employment.The public commitment to disclose severe risks✱PAI’s Shared Prosperity Guidelines use UNGP’s definition of severity: an impact (potential or actual) can be severe “by virtue of one or more of the following characteristics: its scale, scope or irremediability. Scale means the gravity of the impact on the human right(s). Scope means the number of individuals that are or could be affected. Irremediability means the ease or otherwise with which those impacted could be restored to their prior enjoyment of the right(s).” https://www.ungpreporting.org/glossary/severe-human-rights-impact/ should specify the severity threshold considered by the organization to warrant disclosure, as well as explain how the threshold level of severity was chosen and what external stakeholders were consulted in that decision.
Alternatively, an organization can choose to set a threshold in terms of an AI system’s marketed capabilities and disclose all risk signals which are present for systems meeting that threshold. For example, if an organization’s expected return on investment from the use of an AI system under assessment is a multiple greater than 10, its corresponding risks would be subject to disclosure. In instances where organizational impact is driven by a series of smaller system implementations, the organization could choose to disclose all risk signals present once the cumulative cost decrease or revenue increase exceeds 5%.✱A recent study of corporate respondents showed roughly one quarter of respondents were able to achieve a 5% improvement to EBIT in 2021. As AI adoption becomes more widespread, we anticipate more organizations will meet this threshold. https://www.mckinsey.com/capabilities/quantumblack/our-insights/the-state-of-ai-in-2022-and-a-half-decade-in-review#/
Institute for the Future of Work, Good Work Algorithmic Impact Assessment
Throughout the entire procurement process, from identification to use
Run opportunity and risk analyses early and often across AI implementation and use, using the data available at each stage. Update as more data becomes available (for example, as objectives are identified, systems are procured, implementation is completed, and new applications arise). Whenever applicable, we suggest using AI system implementation and use choices to maximize the presence of signals of opportunity and minimize the presence of signals of risk.Solicit the input of workers that stand to be affected✱It is frequently the case that workers who stand to be affected by the introduction of an AI system include not only workers directly employed by the organization introducing AI in its own operations, but a wider set of current or potential labor market participants. Therefore it is important that not only incumbent workers are given the agency to participate in job impact assessment and risk mitigation strategy development. and a multi-disciplinary set of independent experts when assessing the presence of opportunity and risk signals. Make sure to compensate external contributors for their participation in the assessment of the AI system.
Please note that the analysis of opportunity and risk signals suggested here is different from red team analysis suggested in RPU15. The former identifies risks and opportunities created by an AI system working perfectly as intended. The latter identifies possible harms if the AI system in question malfunctions or is misused.
In alignment with UN Guiding Principles for Business and Human Rights, a mitigation strategy should be developed for each risk identified, prioritizing the risks primarily by severity of potential impact and secondarily by its likelihood. Severity and likelihood of potential impact are determined on a case-by-case basis.✱An algorithm described here is very useful for determining the severity of potential quantitative impacts (such as impacts on wages and employment), especially in cases with limited uncertainty around the future uses of the AI system being assessed: https://www.brookings.edu/research/how-innovation-affects-labor-markets-an-impact-assessment/Korinek, A. (2022). How innovation affects labor markets: An impact assessment.Mitigation strategies can range from eliminating the risk or reducing the severity of potential impact to ensuring access to remedy or compensation for affected groups. If effective mitigation strategies for a given risk are not available, this should be considered a strong argument in favor of meaningful changes in the development plans of an AI system, especially if it is expected to affect vulnerable groups.
Engaging workers and external experts as needed in the creation of mitigation strategies is critical to ensure important considerations are not being missed. It is especially critical to engage with representatives of communities that stand to be affected. Please ensure that everyone engaged in consultations around assessing risks and developing mitigation strategies is adequately compensated.
Workers who will use or be affected by AI hold unique perspectives on important needs and opportunities in their roles. They also possess particular insight into how AI systems could create harm in their workplaces. To ensure AI systems foster shared prosperity, these workers should be included and afforded agency in the AI procurement, implementation, and use process from start to finish.Institute for the Future of Work. (2023). Good Work Algorithmic Impact Assessment Version 1: An approach for worker involvement. https://tinyurl.com/mr4yn5ytWorkers must be properly equipped with knowledge of potential product functions, capabilities, and limitations, so that they can draw meaningful connections to their role-based knowledge (see RPU13 for more information). Additionally, care must be taken to create a shared vocabulary on the team, so that technical terms or jargon do not unintentionally obscure or mislead. Workers must also be given genuine decision-making power in the process, allowing them to shape use (such as new workflows or job design) and be taken seriously on the need to end a project if they identify unacceptable harms that cannot be resolved.
AI systems are less likely to cause harm in environments with:
- High levels of legal protection, monitoring, and enforcement for workers’ rights (such as those related to health and safety or freedom to organize)
- High levels of worker voice and negotiating ability (due to strong protections for worker voice or high demand for workers’ comparatively scarce skills), especially those where workers have meaningful input into decisions regarding the introduction of new technologies
These factors encourage worker-centric AI design. Workers in such environments also possess a higher ability to limit harms from AI systems (such as changing elements of an implementation or rejecting the use of the technology as needed), including harms outside direct legal protections. This should not, however, be treated as a failsafe for harmful technologies: other practices in this list should also be followed to reduce risk to workers.
Key requirements for the responsible sourcing of data enrichment services (such as, data annotation and real-time human verification of algorithmic predictions) include:
- Always paying data enrichment workers above the local living wage
- Providing clear, tested instructions for data enrichment tasks
- Equipping workers with simple and effective mechanisms for reporting issues, asking questions, and providing feedback on the instructions or task design
In collaboration with our Partners, PAI has developed a library of practitioner resources for responsible data enrichment sourcing.
When identifying needs, procuring, and implementing AI systems
AI systems welcomed by workers largely fall into three overarching categories:
- Systems that directly improve some element of job quality
- Systems that assist workers to achieve higher performance on their core tasks
- Systems that eliminate undesirable non-core tasks (See OS2, OS9, RS1, and RS2 for additional detail)
Starting with one of these objectives in mind and creating robust participation mechanisms for workers throughout the design and implementation process is likely to result in win-win-wins for AI creators, employers who implement AI, and the workers who use or are affected by them.
As discussed throughout, AI systems raise substantial concerns about the risks of their adoption in workplace settings. To understand and address these risks, experts are needed to vet and implement AI systems. In addition to technical experts, this includes sociotechnical experts capable of performing the Job Impact Assessment described above to the level of granularity necessary to fully identify and mitigate risks of a specific system in a given workplace.The importance of this practice increases with AI system customization or integration. In situations where systems are developed by organizations who follow the Shared Prosperity Guidelines or similar recommendations, disclose potential labor impacts, and design these systems to be used off-the-shelf, less internal expertise may be required from users. However, when systems are more customized or integrated into workplaces, specifics related to the organization and worksite more heavily influence labor impacts arising from the particulars of system use, requiring additional expertise.
Privacy and ownership over data generated by one’s activities are increasingly rights recognized inside and outside the workplace. Respect for these rights requires fully informing workers about the data collected on them and inferences made, how they are used and why, as well as offering them the ability to opt out of collection and use.Bernhardt, A., Suleiman, R., and Kresge, L. (2021). Data and algorithms at work: the case for worker technology rights. https://laborcenter.berkeley.edu/wp-content/uploads/2021/11/Data-and-Algorithms-at-Work.pdf. Workers should also be given the opportunity to individually or collectively forbid the sales of datasets that include their personal information or personally identifiable information. Depending on use, generative AI may present novel privacy risks, through extracting information about worker practices and sharing with managers and colleagues. System design and use should follow the data minimization principle: collect only the necessary data, for the necessary purpose, and hold it only for the necessary amount of time. Design should also enable workers to know about, correct, or delete inferences about them.Colclough, C.J. (2022). Righting the Wrong: Putting Workers’ Data Rights Firmly on the Table. https://tinyurl.com/26ycnpv2Particular care must be taken in workplaces, as the power imbalance between employer and employee undermines workers’ ability to freely consent to data collection and use compared to other, less coercive contexts. In practice, data use decisions by employers often shift over time, making it especially important for AI-using organizations to explicitly and transparently inform workers regarding each new use of their data and its implications, and request consent for each new use or repurposing.Brand, J., Dencik, L. and Murphy, S. (2023). The Datafied Workplace and Trade Unions in the UK. Data Justice Lab. https://datajusticeproject.net/wp-content/uploads/sites/30/2023/04/Unions-Report_final.pdf.
Shared Prosperity Guidelines Home
Get Involved
Partnership on AI needs your help to refine, test, and drive adoption of the Shared Prosperity Guidelines.
Fill out the form below to share your feedback on the Guidelines, ask about collaboration opportunities, and receive updates about events and other future work by the AI and Shared Prosperity Initiative.