Risks to Communities
Risks to Communities
Expanding Surveillance Infrastructure in the Pursuit of Fairness
As discussed throughout this paper, there is often a trade-off between privacy and fairness when it comes to assessing discrimination and inequality. Zooming out from the scale of the individual to the scale of communities and groups of people, demographic data collection runs the risk of relying on, and justifying the expansion of, surveillance infrastructures. Scholars of surveillance and privacy have shown time and time again that the most disenfranchised and “at-risk” communities are routinely made “hypervisible” by being subjected to invasive, cumbersome, and experimental data collection methods, often under the rationale of improving services and resource allocation Benjamin, R. (2019). Race after technology: Abolitionist tools for the new Jim code. Polity. Browne, S. (2015). Dark Matters: On the Surveillance of Blackness. In Dark Matters. Duke University Press. https://doi.org/10.1515/9780822375302 Eubanks, V. (2017). Automating Inequality: How High-Tech Tools Profile, Police, and Punish the Poor. St. Martin’s Press..
Within this context of hypervisibility, it is not unreasonable for members of disenfranchised groups to distrust new data collection efforts and to withhold information about themselves when sharing it is optional. As such, it is likely that efforts at demographic data collection result in these groups being underrepresented in datasets. When this has happened in the past, well-meaning practitioners have sought to improve representation and system performance for these groups, motivating more targeted data collection efforts without much regard for the burdens and risks of this type of inclusion Hoffmann, 2020.
In cases where there seems to be a tradeoff between institutional visibility or anti-discrimination and surveillance, we recommend centering the agency of the groups that planned interventions are supposed to support. Scholarship from the emerging fields of Indigenous Data Sovereignty and Data Justice can provide a starting point for what this might look like — instead of collecting demographic data to “objectively” or “authoritatively” diagnose a problem in the system or even in society more broadly, data collection efforts can be grounded in community needs and understandings first and foremost Rainie, S. C., Kukutai, T., Walter, M., Figueroa-Rodríguez, O. L., Walker, J., & Axelsson, P. (2019). Indigenous data sovereignty. Ricaurte, P. (2019). Data Epistemologies, Coloniality of Power, and Resistance. Television & New Media, 16. Walter, M. (2020, October 7). Delivering Indigenous Data Sovereignty. https://www.youtube.com/watch?v=NCsCZJ8ugPA.
Misrepresentation and Reinforcing Oppressive or Overly Prescriptive Categories
Another source of risk arises from the demographic categories themselves and what they are taken to represent. Scholars from a wide range of disciplines have considered the question of what constitutes representative or useful categorization schemas for race, gender, sexuality, and other demographics of institutional interest and where there are potential sources for harm See, for example: Bowker, G. C., & Star, S. L. (1999). Sorting things out: Classification and its consequences. MIT Press. See, for example: Dembroff, R. (2018). Real Talk on the Metaphysics of Gender. Philosophical Topics, 46(2), 21–50. https://doi.org/10.5840/philtopics201846212 See, for example: Hacking, I. (1995). The looping effects of human kinds. In Causal cognition: A multidisciplinary debate (pp. 351–394). Clarendon Press/Oxford University Press. See, for example: Hanna et al., 2020 See, for example: Hu, L., & Kohler-Hausmann, I. (2020). What’s Sex Got to Do With Fair Machine Learning? 11. See, for example: Keyes (2019) See, for example: Zuberi, T., & Bonilla-Silva, E. (2008). White Logic, White Methods: Racism and Methodology. Rowman & Littlefield Publishers.. Though there are certainly nuances to defining and measuring each of these demographics, we can find some general trends across this scholarship around the risks of uncritically relying on these categories to describe the world, or, in our case, to ascertain system treatment across groups. At a high level, these risks center around essentializing or naturalizing schemas of categorization, categorizing without flexibility over space and time, and misrepresenting reality by treating demographic categories as isolated variables instead of “structural, institutional, and relational phenomenon.” Hanna et al., 2020
Demographic data collection efforts can reinforce oppressive norms & the delegitimization of disenfranchised groups.
The first of these risks, and certainly the one most frequently encountered and vocalized by practitioners Andrus et al., 2021, is when entire groups are forced into boxes that do not align with or represent their identity and lived experience. Often, this occurs because the range of demographic categories is too narrow, such as leaving out options for “non-binary” or “gender-fluid” in the case of gender Bivens, 2017. It can also commonly occur in cases where demographic data is collected through inference or ascription by someone other than the data subject themselves. In these cases, systems often embed very narrow standards for what it means to be part of a group, defining elements of identity in a way that does not align with the experience of entire segments of the population. This type of risk is especially well-documented with regards to various types of automated gender recognition failing to correctly categorize transgender and non-binary individuals. Both critics and users deem these failures inevitable because these systems treat gender as purely physiological or visual, which is different from how members of these communities actually experience gender Hamidi, F., Scheuerman, M. K., & Branham, S. M. (2018). Gender Recognition or Gender Reductionism?: The Social Implications of Embedded Gender Recognition Systems. Proceedings of the 2018 CHI Conference on Human Factors in Computing Systems – CHI ’18, 1–13. https://doi.org/10.1145/3173574.3173582 Keyes, 2018 Keyes, 2021. In both of these ways, demographic data collection efforts can reinforce oppressive norms and the delegitimization of disenfranchised groups, potentially excluding entire communities from services and institutional recognition as a form of what critical trans scholar Dean Spade (2015) calls “administrative violence.”
Furthermore, data collected with overly narrow categories risks misrepresenting and obscuring subgroups subject to distinct forms of discrimination and inequality. This is often the case with Hmong communities which, despite facing extreme disenfranchisement, are seen as having opportunities typical of the larger AAPI category Fu, S., & King, K. (2021). Data disaggregation and its discontents: Discourses of civil rights, efficiency and ethnic registry. Discourse: Studies in the Cultural Politics of Education, 42(2), 199–214. https://doi.org/10.1080/01596306.2019.1602507 Poon et al., 2017.
Another way that categorization schema can be misaligned with various groups’ experiences and lived realities is when the demographic variables themselves are too narrowly defined to capture all the dimensions of possible inequality. For example, as previously discussed, race can be self-identified (how an individual sees themselves), ascribed (how others see them), or relational (variable with regards to who or what someone is interacting with), and each of these dimensions carries with it different potential adverse treatments and effects Hanna et al., 2020. If the only type of demographic data an institution collects is through self-identification, for instance, it can draw a very different picture of discrimination than data collected through ascription Saperstein, A. (2012). Capturing complexity in the United States: Which aspects of race matter and when? Ethnic and Racial Studies, 35(8), 1484–1502. https://doi.org/10.1080/01419870.2011.607504. As such, when it comes to assessing discrimination or some other form of inequality, it is critical that practitioners have a prior understanding of how differential treatment or outcomes are likely to occur such that the right dimension of identity is captured to accurately assess likely inequities.*
Finally, it is important to consider the temporality of categorization — categorization schema and identities can change over time, and how much this is taken into account during system design will likely have a disproportionate impact on groups with more fluidity in their identities. Looking first to gender and sexuality, critical data scholars have argued that queer and trans identities are inherently fluid, contextual, and reliant upon individual autonomy Keyes, 2019 Ruberg, B., & Ruelos, S. (2020). Data for queer lives: How LGBTQ gender and sexuality identities challenge norms of demographics. Big Data & Society, 7(1), 2053951720933286. https://doi.org/10.1177/2053951720933286. There are no tests or immutable standards for what it means to be queer, non-binary, or any number of other forms of identity, and it is likely that one’s presentation will change over time given new experiences and contexts. In other words, queer identities can be seen as perpetually in a state of becoming, such that, rigid, persistent categorizations into states of being can actually be antithetical to these identities. Pushing towards actionable interventions, Tomasev et al. (2021) Tomasev et al., 2021 suggest moving past attempts to more accurately label queer individuals and groups as a way of achieving fairness and looking instead to qualitatively engage with queer experiences with platforms and services to see how cisheteronormativity crops up in system design.
Somewhat similarly, in studies of race it has been argued that race can (and often should be) seen as a “a dynamic and interactive process, rather than a fixed thing that someone has.” (Pauker et al., 2018) Especially for multiracial individuals, there is immense malleability in how they are perceived by others, how they perceive themselves, and what they choose to accentuate in their presentation and interactions to influence various forms of racial classification Pauker et al., 2018. Similar to the case with queer identities, attempts to come up with and enforce fairness constraints around more static, decontextualized notions of race will miss the ways in which forcing groups into static boxes is itself a form of unfairness. As such, when it is not possible to work with these fluid identity groups directly to understand how systems fail to accommodate their fluidity and mistreat them through other means, data subjects should at the very least be given opportunities to update or clarify their demographics in cases where data is collected over an extended period of time and it is used in variable contexts Ruberg & Ruelos, 2020.
Even in cases where groups feel adequately represented by a categorization schema, however, the categories can become harmful depending on how they are used. When demographic categories start to form the basis for differences in servicing, such as in advertising and content recommendation, there is a risk of reinforcing and naturalizing the distinctions between groups. Especially in cases where demographic variables are uncritically adopted as an axis for differential analysis, varying outcomes across groups can be incorrectly attributed to these variables, as has occurred many times in medical research Braun, L., Fausto-Sterling, A., Fullwiley, D., Hammonds, E. M., Nelson, A., Quivers, W., Reverby, S. M., & Shields, A. E. (2007). Racial Categories in Medical Practice: How Useful Are They? PLOS Medicine, 4(9), e271. https://doi.org/10.1371/journal.pmed.0040271, which in turn reinforces the notion that the differences between groups are natural and not a result of other social factors Hanna et al., 2020. With regards to race, a categorization schema that is conclusively not genetic or otherwise biological Morning, A. (2014). Does Genomics Challenge the Social Construction of Race?: Sociological Theory. https://doi.org/10.1177/0735275114550881, this has been described as the risk of studying race instead of racism. By looking for differences between what groups do instead of how groups are treated, it encourages attributing responsibility to oppressed groups for their own oppression. For example, in the creation of recidivism risk scores tools for the criminal justice system, there has been extensive focus on what factors increase the accuracy of criminality prediction Barabas, C. (2019). Beyond Bias: Re-Imagining the Terms of ‘Ethical AI’ in Criminal Law. SSRN Electronic Journal. https://doi.org/10.2139/ssrn.3377921. However, given how criminality is usually defined — namely, that an individual has been arrested and charged for a crime — the factors that end up predicting criminality most accurately are often just the factors that increase one’s likelihood to be targeted by discriminatory policing Barabas, 2019.
Another way that risks can arise through relatively accurate categorization schema is through what philosopher Ian Hacking (1995) Hacking, 1995 refers to as a “looping effect” between categorization schema and a group’s interaction with the world. As individuals come to understand the differences that form the basis for categorization, they can start to interpret their own actions through the lens of the category they are assigned to, in turn influencing their future decisions. This effect is most often looked at in the context of psychiatric diagnostics, where individuals given a certain diagnosis start to adhere more closely to the diagnostic criteria over time, intentionally or not Hacking, 1995. That being said, it is also applicable to other types of categories as well, such as gender and sexuality Dembroff, 2018. When individuals are made more acutely aware of what factors lead to them being perceived as “a woman” or as “queer,” they are incentivized to change their behavior either to increase the likelihood of their preferred classification or to simply live in a way they may now see as more aligned with their identity. Though this type of risk is not likely to be the most salient when collecting demographic data only to assess unequal outcomes or treatment, it is extremely important to consider when building systems that become increasingly tailored to users based on the information they provide, such as in the case of content recommendation algorithms as used by YouTube and TikTok.
Private Control Over Scoping Bias and Discrimination
As a final risk to consider, the assessment of inequality and discrimination is a not rigidly defined or widely agreed upon process. Rather, institutions that collect demographic data have a wide range of techniques and approaches they can possibly employ when it comes to both collecting data and interpreting that data. As such, if we are asking already marginalized groups to share information for the purposes of assessing unfairness, it is imperative that the institution in question operationalizes fairness in a way that is aligned with these groups’ interests and that we collect data that allows us to construct an accurate representation of the way members of these groups interact with systems.
Attempts to be neutral or objective often have the effect of reinforcing the status quo.
In determining what standards of fairness an institution is likely to use, it can be instructive to consider the institution’s motivations for conducting measurements of fairness in the first place. Though there are many reasons an institution might try to assess and mitigate discrimination and inequalities in their machine learning and algorithmic decision-making systems, much of this work is motivated at least in part by concerns around liability Andrus et al., 2021 Holstein, K., Vaughan, J. W., Daumé III, H., Dudík, M., & Wallach, H. (2019). Improving fairness in machine learning systems: What do industry practitioners need? Proceedings of the 2019 CHI Conference on Human Factors in Computing Systems – CHI ’19, 1–16. https://doi.org/10.1145/3290605.3300830 Rakova, B., Yang, J., Cramer, H., & Chowdhury, R. (2021). Where Responsible AI meets Reality: Practitioner Perspectives on Enablers for shifting Organizational Practices. ArXiv:2006.12358 (Cs). https://doi.org/10.1145/3449081. Generally speaking, however, legal notions of discrimination and fairness remain somewhat limited, often esteeming “neutral” decision-making that attempts to treat everyone the same way as the path towards equality Wachter, S., Mittelstadt, B., & Russell, C. (2021). Why Fairness Cannot Be Automated: Bridging the Gap Between EU Non-Discrimination Law and AI. Computer Law & Security Review, 41. https://doi.org/10.2139/ssrn.3547922 Xenidis, R. (2021). Tuning EU Equality Law to Algorithmic Discrimination: Three Pathways to Resilience. Maastricht Journal of European and Comparative Law, 27, 1023263X2098217. https://doi.org/10.1177/1023263X20982173 Xiang, A. (2021). Reconciling legal and technical approaches to algorithmic bias. Tennessee Law Review, 88(3).. As such, most deployed methods in the algorithmic fairness space are geared towards “de-biasing” decision-making to make it more neutral, rather than trying to directly achieve equality, equity, or another form of social justice Balayn & Gürses, 2021. Given disparate starting points for disenfranchised groups, however, this view that neutrality can lead to a more equal world is both risky and unrealistic, as attempts to be neutral or objective often have the effect of reinforcing the status quo Fazelpour, S., & Lipton, Z. C. (2020). Algorithmic Fairness from a Non-ideal Perspective. Proceedings of the AAAI/ACM Conference on AI, Ethics, and Society, 57–63. https://doi.org/10.1145/3375627.3375828 Green & Viljoen, 2020. Despite this, commitments to neutrality remain the norm for many governmental and corporate policies.
Another element of most technical approaches to fairness measurement is that they are strictly formalized. Formalizability refers to the degree to which it is possible to represent a definition of fairness through mathematical or statistical terms — for instance, defining fairness as correctly categorizing individuals from different groups at the same rate (i.e. true positive parity) is distinctly formalizable. Formalizability is an important attribute of fairness when it has to also coincide with the system design values of efficiency and scalability, because formalization enables a system designer to treat many different problems (e.g. racism, sexism, ableism) similarly. That being said, it also relies on treating much of the world as static. As Green and Viljoen (2020) Green, B., & Viljoen, S. (2020). Algorithmic realism: Expanding the boundaries of algorithmic thought. Proceedings of the 2020 Conference on Fairness, Accountability, and Transparency, 19–31. https://doi.org/10.1145/3351095.3372840 have argued, by treating the point of decision-making as the only possible site of intervention (i.e. adjusting predictions to adhere to some notion of fairness), these attempts at formalization hold fixed many of the engines of discrimination, such as the ways in which different groups interact with institutions and why differences might exist between groups in the first place.
Just as defining fairness, discrimination, or bias is impacted by an institution’s goals and values, the collecting, processing, and interpreting of data is never truly objective. In other words, data is never “raw” because it is shaped by the conditions in which it was collected, the methods that were used, and the goals of measuring the world in the first place Gitelman, L. (2013). Raw Data Is an Oxymoron. MIT Press.. This brings up a salient source of risk in the collection of demographic data: the types of discrimination and inequality that can be assessed using demographic data are largely determined by what other types of data are being collected. For instance, it might be possible to detect that a risk score recidivism tool has unequal outcomes for members of different groups, but without accurate data about interactions between suspects and police and defendants and prosecutors and judges, it may not be possible to accurately assess why these inequalities show up in the data and thus how to best address them Barabas, C., Doyle, C., Rubinovitz, J., & Dinakar, K. (2020). Studying Up: Reorienting the study of algorithmic fairness around issues of power. 10.. Given that data collection efforts must be consciously designed, data always reflects one viewpoint or another about what is important to understand about the world. When those collecting data have blindspots about what impacts decision-making and individuals’ life experiences, various forms of discrimination and inequality run the risk of being misread as inherent qualities of groups or cultural differences between them Crooks, R., & Currie, M. (2021). Numbers will not save us: Agonistic data practices. The Information Society, 0(0), 1–19. https://doi.org/10.1080/01972243.2021.1920081. Historian Khalil Gibran Muhammad (2019) Muhammad, K. G. (2019). The Condemnation of Blackness: Race, Crime, and the Making of Modern Urban America, With a New Preface. Harvard University Press. has argued that the seemingly objective focus on data and statistical reasoning has replaced more explicitly racist understandings of racial difference, a shift made possible by the collection and analysis of disaggregated data.
Taking these subjectivities of fairness measurement into account, there is a significant risk that the collection of demographic data enables private entities to selectively tweak their systems and present them as fair without meaningfully improving the experience of marginalized groups. So long as the data used to assess fairness is collected and housed by private actors, these actors are given substantial agency in scoping what constitutes fair decision-making going forward. One striking example of this already occurring is the creation and normalization of “actuarial fairness,” or that “each person should pay for his own risk,” in the insurance industry Ochigame, R., Barabas, C., Dinakar, K., Virza, M., & Ito, J. (2018). Beyond Legitimation: Rethinking Fairness, Interpretability, and Accuracy in Machine Learning. International Conference on Machine Learning, 6.. Using statistical arguments about the uneven distribution of risk across different demographic categories, industry professionals were able to make the case for what previously might have been considered outright discrimination — charging someone more for insurance because their immutable demographic attributes statistically increase their risk Ochigame et al., 2018. To potentially mitigate some of this risk, institutions looking to collect demographic data should include more explicit documentation and commitments around what types of changes they are looking to make through assessing and bias and discrimination.
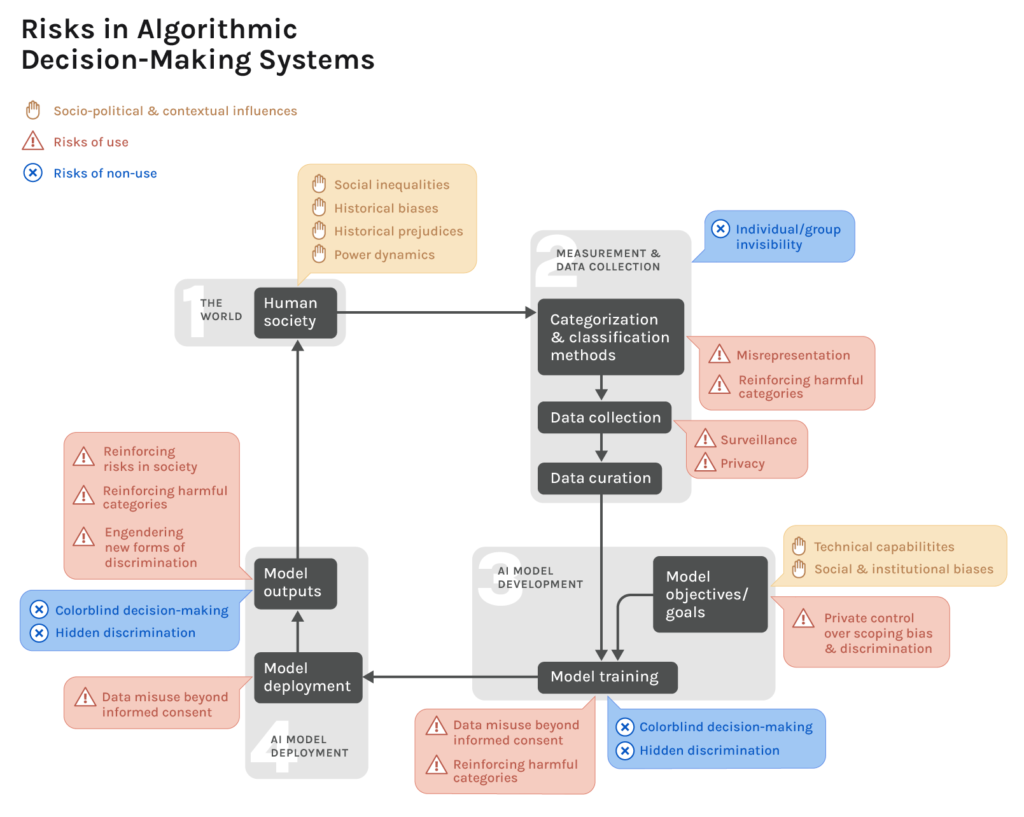