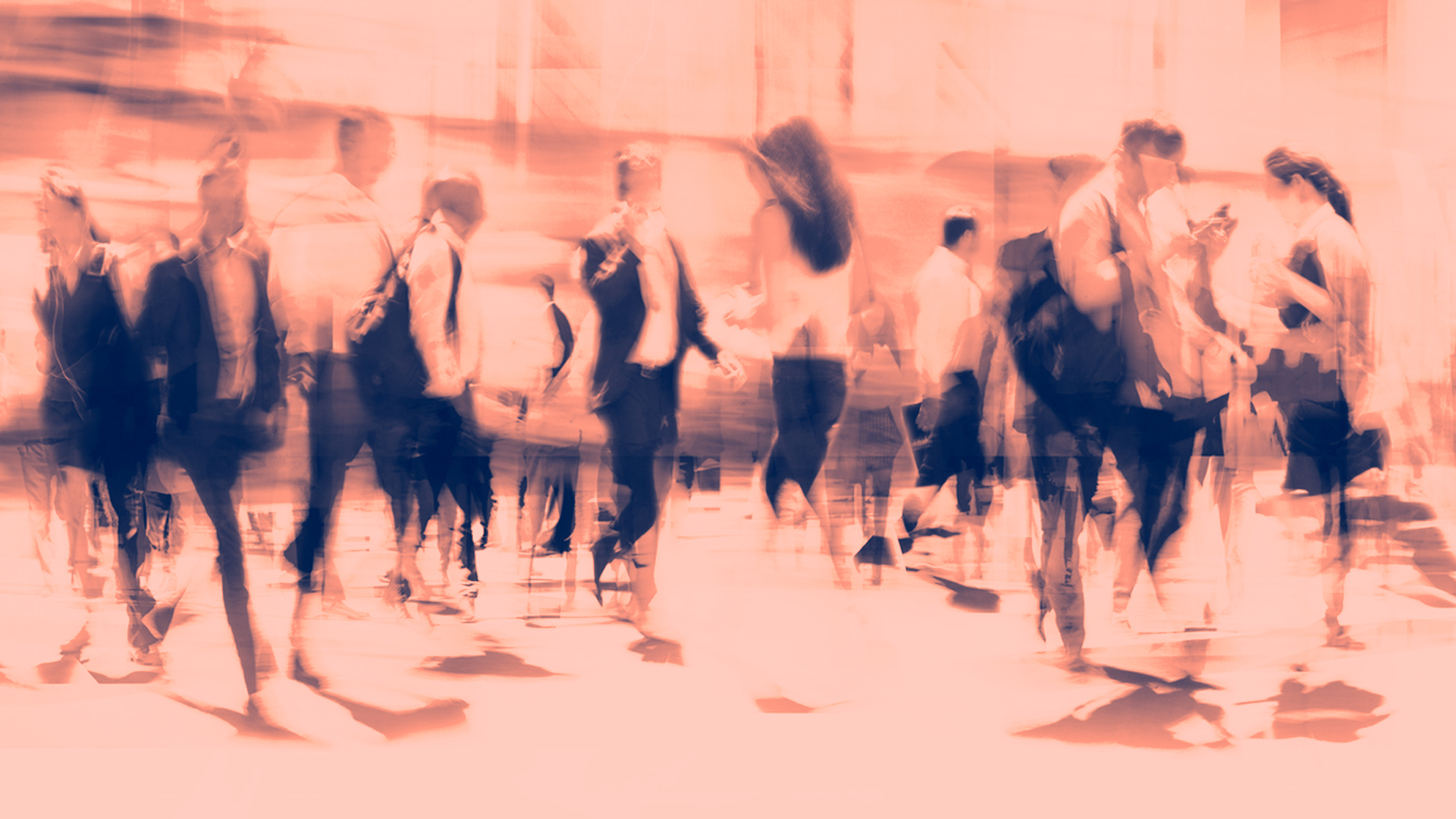
Framework for Promoting Workforce Well-being in the AI-Integrated Workplace
Executive Summary
Executive Summary
The Partnership on AI’s “Framework for Promoting Workforce Well-being in the AI- Integrated Workplace” provides a conceptual framework and a set of tools to guide employers, workers, and other stakeholders towards promoting workforce well-being throughout the process of introducing AI into the workplace.
As AI technologies become increasingly prevalent in the workplace, our goal is to place workforce well-being at the center of this technological change and resulting metamorphosis in work, well-being, and society, and provide a starting point to discuss and create pragmatic solutions.
The paper categorizes aspects of workforce well-being that should be prioritized and protected throughout AI integration into six pillars. Human rights is the first pillar, and supports all aspects of workforce well-being. The five additional pillars of well-being include physical, financial, intellectual, emotional well-being, as well as purpose and meaning. The Framework presents a set of recommendations that organizations can use to guide organizational thinking about promoting well-being throughout the integration of AI in the workplace.
The Framework is designed to initiate and inform discussions on the impact of AI that strengthen the reciprocal obligations between workers and employers, while grounding that discourse in six pillars of worker well-being.
We recognize that the impacts of AI are still emerging and often difficult to distinguish from the impact of broader digital transformation, leading to organizations being challenged to address the unknown and potentially fundamental changes that AI may bring to the workplace. We strongly advise that management collaborate with workers directly or with worker representatives in the development, integration, and use of AI systems in the workplace, as well as in the discussion and implementation of this Framework.
We acknowledge that the contexts for having a dialogue on worker well-being may differ. For instance, in some countries there are formal structures in place such as workers’ councils that facilitate the dialogue between employers and workers. In other cases, countries or sectors do not have these institutions in place, nor a tradition for dialogue between the two parties. In all cases, the aim of this Framework is to be a useful tool for all parties to collaboratively ensure that the introduction of AI technologies goes hand in hand with a commitment to worker well-being. The importance of making such commitment in earnest has been highlighted by the COVID-19 public health and economic crises which exposed and exacerbated the long-standing inequities in the treatment of workers. Making sure those are not perpetuated further with the introduction of AI systems into the workplace requires deliberate efforts and will not happen automatically.
Recommendations
Recommendations
This section articulates a set of recommendations to guide organizational approaches and thinking on what to promote, what to be cognizant of, and what to protect against, in terms of worker and workforce well-being while integrating AI into the workplace. These recommendations are organized along the six well-being pillars identified above, and are meant to serve as a starting place for organizations seeking to apply the present Framework to promote workforce well-being throughout the process of AI integration. Ideally, these can be recognized formally as organizational commitments at the board level and subsequently discussed openly and regularly with the entire organization.
The “Framework for Promoting Workforce Well-Being in the AI-Integrated Workplace” is a product of the Partnership on AI’s AI, Labor, and the Economy (AILE) Expert Group, formed through a collaborative process of research, scoping, and iteration. In August 2019, at a workshop called “Workforce Well-being in the AI-Integrated Workplace” co-hosted by PAI and the Ford Foundation, this work received additional input from experts, academics, industry, labor unions, and civil society. Though this document reflects the inputs of many PAI Partner organizations, it should not under any circumstances be read as representing the views of any particular organization or individual within this Expert Group, or any specific PAI Partner.
Acknowledgements
The Partnership on AI is deeply grateful for the input of many colleagues and partners, especially Elonnai Hickok, Ann Skeet, Christina Colclough, Richard Zuroff, Jonnie Penn as well as the participants of the August 2019 workshop co-hosted by PAI and the Ford Foundation. We thank Arindrajit Basu, Pranav Bidaire, and Saumyaa Naidu for the research support.