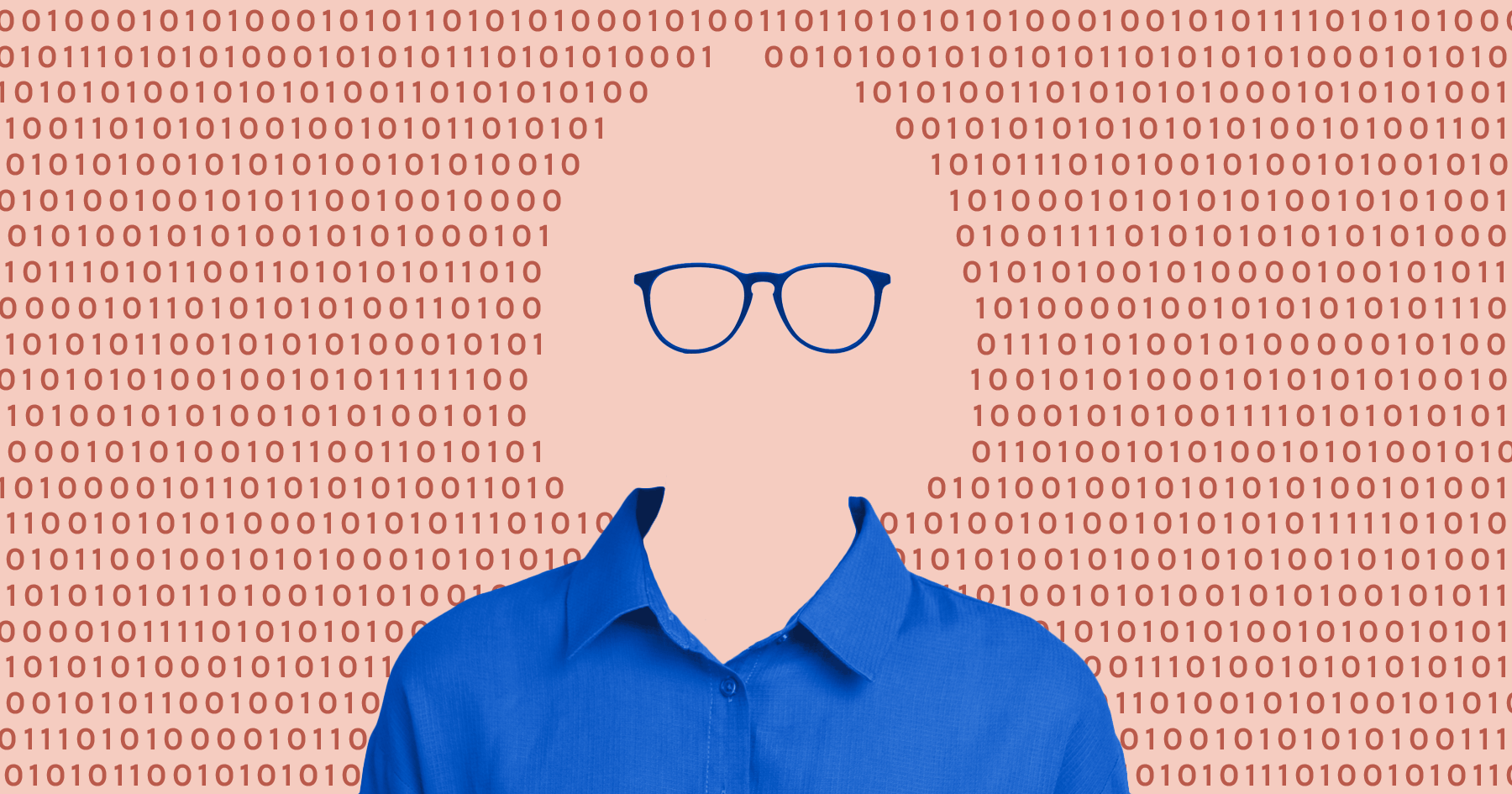
Eyes Off My Data: Exploring Differentially Private Federated Statistics To Support Algorithmic Bias Assessments Across Demographic Groups
Executive Summary
Executive Summary
Designing and deploying algorithmic systems that work as expected every time for all people and situations remains a challenge and a priority. Rigorous pre- and post-deployment fairness assessments are necessary to surface any potential bias in algorithmic systems. As they often involve collecting new user data, including sensitive demographic data, post-deployment fairness assessments to observe whether the algorithm is operating in ways that disadvantage any specific group of people can pose additional challenges to organizations. The collection and use of demographic data is difficult for organizations because it is entwined with highly contested social, regulatory, privacy, and economic considerations. Over the past several years, Partnership on AI (PAI) has investigated key risks and harms individuals and communities face when companies collect and use demographic data. In addition to well-known data privacy and security risks, such harms can stem from having one’s social identity being miscategorized or data being used beyond data subjects’ expectations, which PAI has explored through our demographic data workstream. These risks and harms are particularly acute for socially marginalized groups, such as people of color, women, and LGBTQIA+ people.
Given these risks and concerns, organizations developing digital technology are invested in the responsible collection and use of demographic data to identify and address algorithmic bias. For example, in an effort to deploy algorithmically driven features responsibly, Apple introduced IDs in Apple Wallet with mechanisms in place to help Apple and their partner issuing state authorities (e.g., departments of motor vehicles) identify any potential biases users may experience when adding their IDs to their iPhones.*IDs in Wallet, in partnership with state identification-issuing authorities (e.g., departments of motor vehicles), were only available in select US states at the time of the writing of this report.
In addition to pre-deployment algorithmic fairness testing, Apple followed a post-deployment assessment strategy as well. As part of IDs in Wallet, Apple applied differentially private federated statistics as a way to protect users’ data, including their demographic data. The main benefit of using differentially private federated statistics is the preservation of data privacy by combining the features of differential privacy (e.g., adding statistical noise to data to prevent re-identification) and federated statistics (e.g., analyzing user data on individual devices, rather than on a central server, to avoid the creation and transfer of datasets that can be hacked or otherwise misused). What is less clear is whether differentially private federated statistics can attend to some of the other risks and harms associated with the collection and analysis of demographic data. To understand this, a sociotechnical lens is necessary to understand the potential social impact of the application of a technical approach.
This report is the result of two expert convenings independently organized and hosted by PAI. As a partner organization of PAI, Apple shared details about the use of differentially private federated statistics as part of their post-deployment algorithmic bias assessment for the release of this new feature.
During the convenings, responsible AI, algorithmic fairness, and social inequality experts discussed how algorithmic fairness assessments can be strengthened, challenged, or otherwise unaffected by the use of differentially private federated statistics. While the IDs in Wallet use case is limited to the US context, the participants expanded the scope of their discussion to consider differential private federated statistics in different contexts. Recognizing that data privacy and security are not the only concerns people have regarding the collection and use of their demographic data, participants were directed to consider whether differentially private federated statistics could also be leveraged to attend to some of the other social risks that can arise, particularly for marginalized demographic groups.
The multi-disciplinary participant group repeatedly emphasized the importance of having both pre- and post-deployment algorithmic fairness assessments throughout the development and deployment of an AI-driven system or product/feature. Post-deployment assessments are especially important as they enable organizations to monitor algorithmic systems once deployed in real-life social, political, and economic contexts. They also recognized the importance of thoughtfully collecting key demographic data in order to help identify group-level algorithmic harms.
The expert participants, however, clearly stated that a secure and privacy-preserving way of collecting and analyzing sensitive user data is, on its own, insufficient to deal with the risks and harms of algorithmic bias. In fact, they expressed that such a technique is not entirely sufficient for dealing with the risks and harms of collecting demographic data. Instead, the convening participants identified key choice points facing AI-developing organizations to ensure the use of differentially private federated statistics contributes to overall alignment with responsible AI principles and ethical demographic data collection and use.
This report provides an overview of differentially private federated statistics and the different choice points facing AI-developing organizations in applying differentially private federated statistics in their overall algorithmic fairness assessment strategies. Recommendations for best practices are organized into two parts:
- General considerations that any AI-developing organization should factor into their post-deployment algorithmic fairness assessment
- Design choices specifically related to the use of differentially private federated statistics within a post-deployment algorithmic fairness strategy
The choice points identified by the expert participants emphasize the importance of carefully applying differentially private federated statistics in the context of algorithmic bias assessment. For example, several features of the technique can be determined in such a way that reduces the efficacy of the privacy-preserving and security-enhancing aspects of differentially private federated statistics. Apple’s approach to using differentially private federated statistics aligned with some of the practices suggested during the expert convenings: the decision to limit the data retention period (90 days), allowing users to actively opt-into data sharing (rather than creating an opt-out model), clearly and simply sharing what data the user will be providing for the assessment, and maintaining organizational oversight of the query process and parameters.
The second set of recommendations surfaced by the expert participants primarily focus on the resources (e.g., financial, time allocation, and staffing) necessary to achieve a level of alignment and clarity on the nature of “fairness” and “equity” AI-developing organizations are seeking for their AI-driven tools and products/features. While these considerations may seem tangential, expert participants emphasized the importance of establishing a robust foundation on which differentially private federated statistics could be effectively utilized. Differentially private federated statistics, in and of itself, does not mitigate all the potential risks and harms related to collecting and analyzing sensitive demographic data. It can, however, strengthen overall algorithmic fairness assessment strategies by supporting better data privacy and security throughout the assessment process.