Overview
As AI systems are deployed across an ever-growing number of domains, the fairness, transparency, and accountability of these systems has become a critical societal concern. This Program examines the intersections between AI and some of humanity’s most fundamental values, addressing urgent questions about algorithmic equity, explainability, responsibility, and inclusion.
Through original research and multistakeholder input, our Fairness, Transparency, and Accountability work asks how AI can build a world that is more (and not less) just than the one that came before it. And by offering actionable resources for implementing transparency at scale, ABOUT ML seeks to operationalize these insights with full-cycle documentation of machine learning systems.
Our Fairness, Transparency, and Accountability & ABOUT ML Work
Fairness, Transparency, and Accountability encompasses PAI’s large body of research and programming around algorithmic fairness, explainability, criminal justice, and diversity and inclusion. In 2020 alone, this work examined the challenges organizations face when they seek to measure and mitigate algorithmic bias using demographic data, provide meaningful explanations to diverse stakeholders, address bias in recidivism risk assessment tools, and build more inclusive AI teams.
With ABOUT ML, PAI is leading a multistakeholder effort to develop guidelines for the documentation of machine learning systems, setting new industry norms for transparency in AI. This means not just identifying the necessary components of transparency, but releasing actionable resources to help organizations operationalize transparency at scale. Developed through an iterative, multistakeholder process, these resources pool the collective efforts and insights of academic researchers, industry practitioners, civil society organizations, and the impacted public.
Updates
Program Workstreams
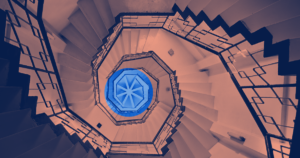
ABOUT ML
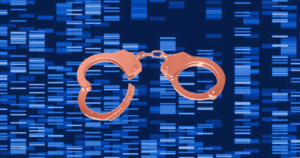
AI & Criminal Justice
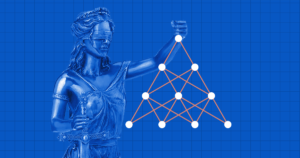
Algorithmic Fairness & the Law
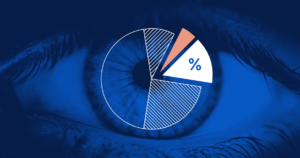