Labeling Misinformation Isn’t Enough. Here’s What Platforms Need to Do Next.
Labeling Misinformation Isn’t Enough. Here’s What Platforms Need to Do Next.
![$hero_image['alt']](https://partnershiponai.org/wp-content/uploads/2021/03/Labeling-Misinformation-Blog02_030921.jpeg)
In the months leading up to last year’s U.S. Presidential election, Facebook labeled more than 180 million posts as misinformation. In the weeks surrounding the election, Twitter flagged about 300,000 more — including several by President Trump himself. As many jump to either celebrate or critique platforms for such actions, a crucial question remains: What are these labels actually doing?
To answer this, we draw on the Partnership on AI and First Draft’s original user research — accepted to 2021 ACM SIGCHI as a Late-Breaking Work paper — which delves into the media habits of internet users across the country, studying their reactions to manipulated media and labeling interventions related to U.S. politics and COVID-19. Although platforms frequently approach misinformation as a challenge they might automate away — one day reaching 100% accuracy in classifying things as “true” or “false” — our findings challenge this basic premise. We discover an American public that is often more concerned with misinformation in the form of editorial slant, rather than wholly fabricated claims and visuals. Our research also reveals a public that is deeply divided about whether to trust social media platforms to determine what’s fake without error or bias in the first place, highlighting the limits of these labels to effectively inform the public when it comes to contentious, politicized issues.
“False information? Says who?”
Across two studies with 34 participants, one of the most frequent criticisms of labels we encountered concerned the role of social media companies. Many believed private platforms simply shouldn’t be rating the accuracy of user-generated content, with almost half of participants reacting to labels with skepticism or outrage. These users, all of whom identified as Republicans or Independents, objected to what they perceived as paternalism and partisan bias. They saw labels as (liberal) platforms spoon-feeding (conservative) users information rather than trusting them to do their own research. Such gut reactions often prevented them from engaging with the actual content of the corrections in good faith.
For example, one Republican participant was a member of a Facebook group called “Partly False Information” where people banded together to mock these labels. He described one flagged post from his feed which, failing to take into account differences in individuals’ risks, misleadingly concluded that the odds of not dying from COVID-19 were 99.96%. Rather than the label persuading him, he viewed it as simply more evidence in line with his prior beliefs that COVID-19 was being exaggerated by the media, fact-checkers, and Facebook itself. “At this point, I just look at it and say, you know what, I have to completely disregard the ‘independent’ fact-checker, because we know that they are so ‘independent,’” he said, gesturing wildly with air quotes. Or, as another Republican participant put it: “It’s sickening how pathetic and in bed social media and the mainstream media is with the Democrats and the Liberals.”
“It was obviously a joke”
Another issue highlighted by our research is the difficulty in properly recognizing nuance and context when processing information at scale. When platforms use hybrid human-AI systems to detect and label millions of posts, it becomes impossible to determine each post’s real intent and the harms it poses to specific communities.
In recent months, platforms have made some efforts to better differentiate between the severity of different types of misinformation and manipulated media. For example, on Facebook and Instagram, when fact-checkers rate a post as “Missing Context” or “Partly False Information” they are labeled with contextual information, whereas more severe ratings like “False Information” and “Altered” use a label that fully covers the post to prevent exposure entirely. Yet, for a given viral misinformation post, fact-checkers don’t have full visibility into the wide variety of context and uses in which their label will apply. What this means is that, in practice, labels don’t always line up with how users understand the actual harm (or lack of harm) a post in their feed poses.
For example, one woman we interviewed, an Independent, described two times she felt labels were applied to her posts without merit. The first post was a meme that she felt “was very obviously a joke.” The second time she knowingly shared a false post, but it was in order to educate others. “I had actually in my post shared this picture and in my own words said ‘this is why you need to fact-check.’ And they still blocked it!” she said. “It’s like they look at the pictures or the headline that you’re posting and not look at what you post with it.” These types of experiences triggered feelings of anger and distrust towards platforms and their corrections.
“This one goes farther in the harm it’s trying to do”
We also found that the labels applied to manipulated media often reflected little about the potential harm the content posed, with a satirical video of Joe Biden’s tongue wagging, a doctored image of shark on a highway, and a photo falsely claiming to show a Gates “Center for Global Human Population Reduction” all treated similarly.
When the more severe intervention of obscuring the post entirely was applied to content seen as more benign, such as the highway shark, many balked. One participant described it as “offensive” because “people are smart enough to understand whether it’s really a shark.” In contrast, the Gates post elicited feedback that an intervention covering the whole post would be beneficial. As one participant put it, “There’s a lot of fake images online that make you want to look, feel an emotion. But this one goes farther in the harm it’s trying to do.” Another added: “Once something is out there, it’s hard to unsee it.”
“I’m tired of divisive news”
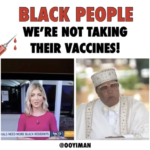
Finally, we found that a focus on labeling specific posts missed the reality that information problems are rooted in complex social dynamics that go far beyond individual false claims. Many participants described feeling exhausted by an overwhelming deluge of conflicting, negative, and polarized opinions on social media. In fact, a lot of misleading narratives pushed by communities like QAnon followers or anti-vaxxers rely on speculation and emotion, not easily falsifiable claims.
Consider one image post addressed to “BLACK PEOPLE” bearing the text: “WE’RE NOT TAKING THEIR VACCINES!” As a general call to action, this is not technically a claim that can be fact-checked. It’s clear that disputing individual false claims and fabricated images will not address the deeper issues of distrust fueling misinformation. And as more users learn how to color within the lines of platform policy to avoid getting labeled or banned, such interventions will be even less effective at preventing the propagation of harmful content by hate groups and conspiracy theorists who can adapt their approaches in real time.
What this means
These examples illustrate why misinformation labels alone are not enough to address information problems online. Even with legions of moderators and advanced AI systems working to identify and label misinformation, claim-based corrections will always resemble a game of whack-a-mole. Additionally, conspiracy theory communities on social media can spread falsehoods at a far greater speed and scale compared to public science communicators and investigative journalists using traditional “top-down” methods. These disinformation networks won’t disappear with a label — and continuing to rely on labels alone risks allowing them to take a greater hold in our digital society.
Do these concerns mean it’s worthless for platforms to try to identify and label misinformation? Not necessarily, but these issues do demand platforms and researchers find ways to better account for the unintended consequences of such actions, and design more effective interventions suited to a variety of people and their distinct information issues.
What platforms can do now
How can we work toward more meaningful misinformation and disinformation interventions for end-users? In a few ways:
1. Release archives of labeled and removed content for researchers.
As long as platforms are in the game of designing processes to classify and act on the credibility of information, they need to provide independent parties sufficient access to evaluate these classifications. Many of our partners in academia and civil society have repeatedly asked platforms for archives and transparency APIs to access flagged and removed information in order to better study this content and make recommendations. For example, researchers Joan Donovan, Gabrielle Lim, WITNESS, and others have proposed the creation of a “human rights locker” that factors in access controls and privacy and security implications of storing such potentially dangerous material.
2. Create tools to enable independent research of interventions using real data and platform environments.
Another challenge in evaluating interventions is that platforms themselves are sitting on literal server farms of behavioral data. While platforms need to protect this personal private data, realistic user context is also crucial to understanding moving misinformation targets. Even as some researchers are finding creative workarounds — such as our diary study method, or opt-in browser projects such as Citizen Browser — ultimately it’s the platforms themselves who are in a position to provide optimal and secure environments for studying what interventions are doing.
3. Draft industry-wide benchmarks and metrics to understand progress and unintended consequences of interventions.
What is an effective intervention? It depends on what you’re measuring and how. Currently, many platforms release transparency reports, but make independent decisions about what data to release in these documents and why. Many of our partners are seeking cross-platform comparisons of processes and intervention effects. How might we make sense of the broader ecosystem-level impacts of interventions and align on what makes an intervention more or less effective in different contexts?
4. Explore approaches that focus on clarifying larger narratives, rather than isolated claims.
Our findings clearly show the limits of focusing on false claims alone. Fortunately, there are a variety of more holistic approaches to explore, such as building in digital literacy and educational interventions, pre-bunking false narratives, or using friction to slow users down and consider the context of posts about controversial subjects. These strategies shift the focus from a whack-a-mole battle to providing users with the confidence and skills to assess claims and information on an ongoing basis.
5. Co-design interventions to meet different people where they are.
As our findings show, reactions toward interventions can vary widely depending on who you ask about them. How might interventions better factor in and center these differences, using entirely different tactics, such as community-centric moderation and participatory co-design of interventions?
On some level, we can’t blame deep social problems on platform action or inaction. There is no way for platforms to ever declare victory in the intractable “war on misinformation.” That is to say, misinformation has always and will always exist in some form. That means the task ahead is not a winnable war, but a dynamic process of understanding the root causes that lead people to engage in misinformation, designing systems to mitigate its harms through interventions tested and refined according to clear societal goals.
As a next step, we intend to work with our partners in civil society, academia, and industry to lead the way on recommendation #3 above, finding common ground about the ideal goals and measures for misinformation interventions. From here, we can better move forward to evaluate when and where platforms’ interventions are effectively bolstering credible information while reducing belief in misinformation.